Machine Learning and How it Is Transforming Transportation
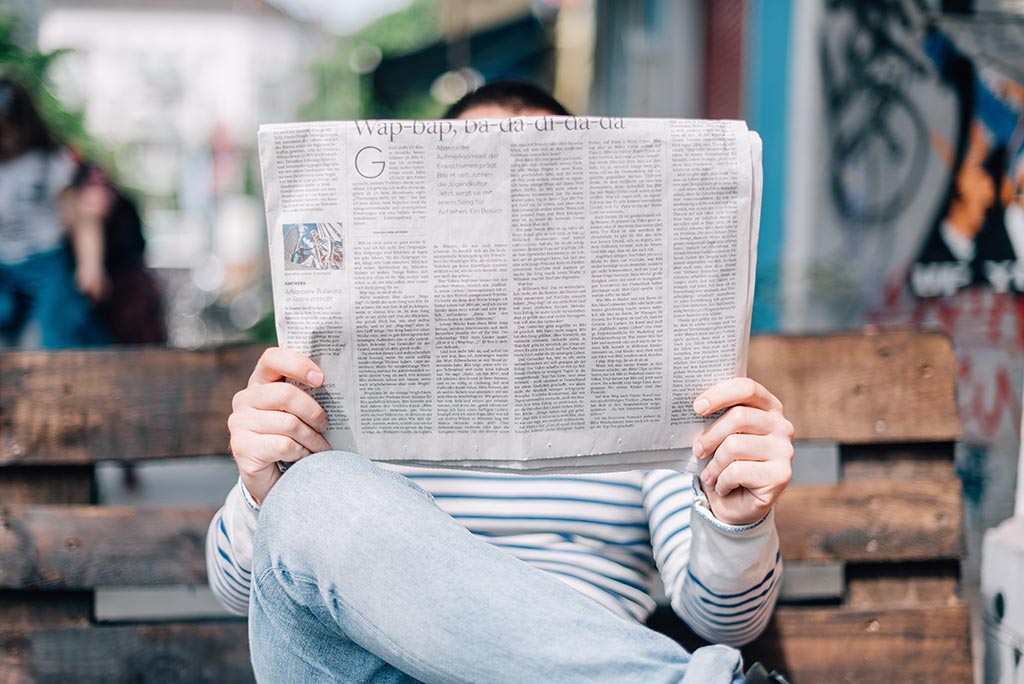
If you are in any way connected to the computer world, you have heard of the term “machine learning”. It is such an important concept, but it has been used as a buzzword so much that it is starting to lose its effectiveness. That said, machine learning is one of the most important developments in the computing world and if it can be utilized to its full potential, it is set to revolutionize the way we use computers. Because of its versatility and flexibility, machine learning can be used in almost any industry where tasks can be automated. These are industries where machines can learn to think like humans and be able to perform at the same level as or even better than humans. One of these areas is transportation.
Much More Than Driving Cars
When many people think about artificial intelligence (AI) and machine learning as it ties into the automotive industry, they think about driverless cars and fleets of cars communicating with each other in real-time. While this is one part of it, there is so much more. Machine learning can be used to:
- Solve congestion in our cities
- Improve the energy efficiency of the cars we drive, thereby helping reduce pollution
- Help plan emergency responses
- Make our roads a lot safer
When doing their research, scientists and computer programmers are starting to look at machine learning at a higher level and using it to revolutionize the engine and to help decision-makers make the best decisions about transportation systems.
A Departure from the Past
In the past, computer programmers had to write code that told the computer what to do in specific situations. This code would get more complex and unmaintainable as computer programmers tried to plan for and provide code for every case their program would encounter. Now, programmers can write the base code and use neural networks to train computers on what to do in all these different scenarios. Because computers are able to crunch data faster than we could, they are able to discover cases we never could.
Now, computer programmers feed machine learning algorithms using:
- Inputs
- Outputs
The machines are then asked to find a relationship between the two. Once it is done, the data produced is used to create modes that are used to make predictions.
The Need for Machine Learning in Transportation
Researchers are using machine learning to explore how transportation systems are designed. This helps them understand what issues are contained therein and how they affect entire transportation systems.
Their research will help transportation departments:
- Identify traffic issues
- Try to solve these issues
- Plan for the future
Understanding the complexity of transportation systems is almost impossible unless researchers comb through a huge amount of data. Machine learning can help them not only decipher this data, but also help them find trends and relationships and see how both of these affect transportation systems.
The insights that come out of such explorations will help:
- Improve the reliability of transportation systems
- Boost productivity
- Save the time and money wasted sitting in traffic
These insights also help with decision making as they can help people and autonomous vehicles make better decisions, help coordinate emergency responses and help planners minimize the impact of the disruption of a transportation system in a given area.
Engine Development and Optimization
Machine learning is also being used to optimize engine designs and the processes used to produce these engines. For example, researchers have been able to develop new combustion models using machine learning. These models have reduced the amount of time it takes to complete engine combustion simulations.
Using neural networks, researchers have also been able to model complex properties that were previously not available. Now, scientists can create complex reaction pathways to see how combustion happens inside new engine models. Because of this, researchers and automotive manufacturers are able to better optimize their engines.
In the past, researchers were forced to reduce the complexity of their combustion models. This is because they did not have powerful tools to help them carry out complex simulations. This led to data that was not as accurate as it should have been. All this has now changed with the advancement of machine learning, deep learning, AI and neural networks.
Optimizing Travel Routes
Computers that run machine learning models are very good at making predictions using past data. This data can be used to optimize route planning for both drivers and fleet managers. Machine learning can help these parties understand:
- All available route options
- How much fuel they will use if they follow different routes
- The environmental impact of following different routes
Once drivers and fleet operators understand all these things, they can choose cars and routes that save fuel while saving time and maximizing transportation efficiency.
Making Transportation Predictions
The only way to understand what is going to happen in the future is to make predictions that are as accurate as possible. This has been enabled by the use of machine learning. Machine learning is being used to predict how transportation systems will look in the future. Researchers are doing this with the aim of predicting the impact of the transportation system on the world around us as it continues to grow and how this growing transportation system will impact energy needs.
Researchers are forced to model their predictions using:
- Vehicle options available today
- The different types of fuel they use
- Alternate modes of transport
Using these predictions, researchers can see how different technologies will impact transportation systems of the future. This allows them to focus on the technologies that will have the most impact.
New Modes of Transport
Machine learning has brought us new modes of transport. These include autonomous cars, driverless shuttles and more. You can click here to learn more about how future transportation is likely to look. Perhaps the most common mode of transportation impacted by machine learning is autonomous cars.
Autonomous cars are fitted with computers that run different scenarios as they drive or are driven around. This computer makes it possible for this car to identify:
- People
- Other cars
- Other types of transportation options like motorcycles
- Roads and their markings
- Curbs
All this data is used to identify the safest route to follow to avoid collisions and keep transportation systems as safe as possible. As it stands, these cars need a human to always be behind the wheel in case of an emergency. As this technology matures and the computers in autonomous cars become more powerful, we will have cars that can drive themselves. The possibilities are both exciting and endless.
Perhaps we will have other autonomous modes of transportation like driverless trucks and autonomous airplanes. At this point, we can only speculate.
The Role of 5G on Future Transportation
It is almost impossible to talk about the future of transportation without talking about 5G technology. 5G is the fifth generation of mobile communication and it comes with so many advantages. The most important of these are:
- Reduced latency (the times it takes to send and receive data between two computers)
- Increased upload and download speeds
As we look into the future of driverless fleets of cars, it becomes clear that these cars need some way to communicate with each other. This is for purposes like overtaking, turning at junctions, giving right of way and more.
Ideally, we want these cars to communicate in real-time or as close to it as we can get. With low latency times and fast speeds, 5G stands as the best option for this purpose. Of course, communication technology will continue to evolve and we might see better speeds and lower latency in the future. That said, we already have something we can use to enable fleets of driverless cars.
The Upsides and Downsides of Machine Learning
Machine learning is a very complex topic, with both upsides and downsides, because we are just starting to see its potential. That said, there are some upsides that we are already seeing:
- Machine learning can help us plan better cities
- ● Machine learning can help drivers and fleets reduce their fuel costs which has a positive impact on the environment
- Machine learning can help emergency response teams do their jobs better by giving them routes that let them reach where they are needed faster
- ●Machine learning will lead to new job opportunities. We are already seeing this as the need for researchers grows and companies look for computer programmers to build machines and deep learning systems for them.
Machine learning has some downsides too. One of the biggest ones is job loss. As machine learning creates jobs in some sectors, it will lead to massive job losses in the transportation sector. Just think about all the drivers who will be left without a job if we switch to driverless cars. All these taxi and long-haul drivers will have to find new jobs.
There is no denying that machine learning is here and it will revolutionize the transportation sector. Its impacts on the reduction of fuel and the time it takes to get from one place to another are touted as its biggest achievements, as is the development of fuel-efficient engines, something that will have a massive positive impact on the environment.