Fujitsu Develops AI Technology to Automatically Differentiate Between Work Tasks in Video Data for Employee Training and Quality Control Purposes
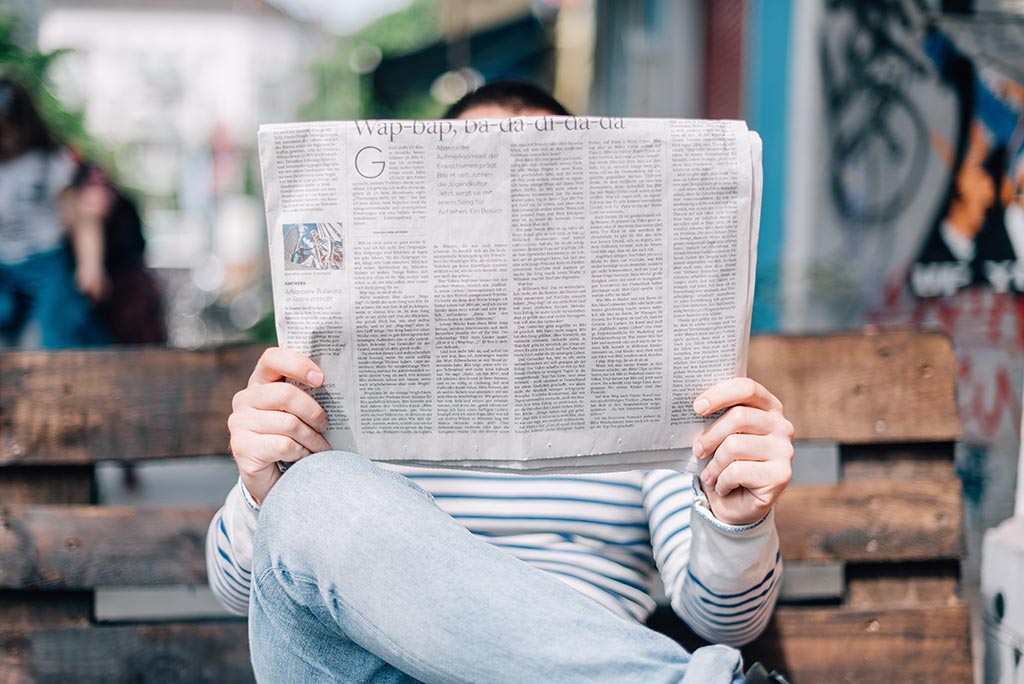
TOKYO, Feb 18, 2021 – (JCN Newswire) – Fujitsu Laboratories Ltd. has announced the development of a technology that automatically detects different work elements–for instance, “removing a part,” “tightening a screw,” or “installing a cover” – from video data consisting of a series of tasks from manufacturing lines and other places of manual work.
|
|
|
Conventionally, in workplaces like factories, efforts to improve productivity and enhance quality have included recording the work of staff and identifying and improving upon problems in different work elements from the data. To measure the time required for each work element from the video data, however, it’s necessary to divide video data into separate work elements manually. This often means that the man-hours required to measure the time for each work element is several times to several tens of times more than the total length of the video.
Fujitsu has expanded upon its “Actlyzer” technology for detecting human movement and behavior from video data, and developed an AI model that takes into account the variation of each movement and the difference in individual workers’ movements, using work data from one person and the data divided by each work element as training data. When this technology was applied to the analysis of operations at Fujitsu I-Network Systems Limited’s Yamanashi Plant (Location: Minami Alps City, Yamanashi Prefecture), it was confirmed that work tasks could be detected with 90% accuracy and that the results could be used to improve the efficiency of operations analysis.
By leveraging this technology, Fujitsu will continue to contribute to the promotion of work process improvement activities and the passing on of specific skills for more efficient work at various sites.
Background
As Japan and many other advanced economies confront declining birthrates, the aging of society, and the increasing mobility of talent, a growing need exists in work that requires human labor to record and analyze vital know-how in the field and use it for creating manuals and offering onsite instruction. As a part of kaizen, or work process improvement, activities aimed at improving productivity and quality at manufacturing sites, many different methods of analysis utilizing video data (Fig.1) are carried out, including determining ways to eliminate waste, comparing work with skilled workers, and measuring the effect of time reduction through streamlining work processes. In these analyses, the work of breaking down video data of different work flows into separate work elements s (Step 2 in Fig.1), including separating the start and end of an element, often takes more than one hour for a 20 minute video, for example. This cumbersome editing process has presented challenges to efforts to accelerate work efficiency and the transmission of skills.
Issues
In recent years, it has become possible to detect the movement of the human skeleton from video images and to recognize common tasks performed by multiple people by training the system using deep learning technologies. In order to achieve this, however, it’s necessary to take into account the subtle variations in movements that occur between individuals even when they perform the same tasks, as well as the differences in movements among different people and environments. This must be done while additionally distinguishing between similar movements and preparing large amounts of training data.
Newly Developed Technology
Fujitsu has successfully developed a technology to automatically detect work elements even for different video data of the same work, taking into account variations in motion and other similar movements, by learning each work element using data obtained by dividing a single video for each element as training data.
First, in order to train the algorithm for different work elements, three-dimensional skeleton recognition technology included in Fujitsu’s behavior analysis technology “Actlyzer”(1) is used to estimate a person’s posture in three-dimensional space from the image that becomes training data. Then, changes in the posture of the upper body are acquired as feature values at intervals of several hundred milliseconds to several seconds, and similar feature values are grouped into several tens of unit movements (m1-mn in Fig.2). In this case, if the characteristics of the change in posture are similar, it is regarded as the same movement, so it is possible to deal with the difference in the way it appears depending on the working position and the position of the camera.
Next, the division position (timestamp) of the work element section corresponding to the training data video is used to associate the discreet movement with the work element. At this time, by estimating the change pattern of the combination of discreet unit movements that constitute the work element and generating it as an AI model, it is possible to absorb the difference in the movement of individuals and the variations in the fine movement that occurs every time even when the same work element is performed. In addition, the AI model automatically recognizes work elements from the sequence of unit movements. This makes it possible to find the work element that best takes into account the overall order of work, even if the same work element exists more than once, such as “tighten a screw”.
Outcomes
At the Yamanashi Plant of Fujitsu I-Network Systems, which manufactures network equipment products, Fujitsu applied the developed technology to analyze the following three work processes (Fig.3): parts setting, assembly, and visual inspection, and conducted an evaluation.
In each process, by only training with the division data of each work element of one person’s video, the work element is automatically detected even in another video of the same work with 90% or more accuracy. As a result, the cycle of kaizen activities can be repeated more frequently, helping to improve work efficiency and accelerate the transfer of skills.
Future Plans
In addition to the manufacturing industry, Fujitsu will continue to verify this technology in various use case scenarios, including logistics, agriculture, and medical care, with the aim of putting it into practical use within fiscal 2021.
(1) behavioral analysis technology “Actlyzer”
Deep learning technology that can recognize a variety of subtle and complex human movements without relying on large amounts of training data. (Press Release: 2019/11/25)
About Fujitsu
Fujitsu is the leading Japanese information and communication technology (ICT) company offering a full range of technology products, solutions and services. Approximately 130,000 Fujitsu people support customers in more than 100 countries. We use our experience and the power of ICT to shape the future of society with our customers. Fujitsu Limited (TSE:6702) reported consolidated revenues of 3.9 trillion yen (US$35 billion) for the fiscal year ended March 31, 2020. For more information, please see www.fujitsu.com.
About Fujitsu Laboratories
Founded in 1968 as a wholly owned subsidiary of Fujitsu Limited, Fujitsu Laboratories Ltd. is one of the premier research centers in the world. With a global network of laboratories in Japan, China, the United States and Europe, the organization conducts a wide range of basic and applied research in the areas of Next-generation Services, Computer Servers, Networks, Electronic Devices and Advanced Materials. For more information, please see: http://www.fujitsu.com/jp/group/labs/en/.
Copyright 2021 JCN Newswire. All rights reserved. www.jcnnewswire.com