In The Race To Use AI, Stop, Think And Take These Five Steps Toward Sustainability
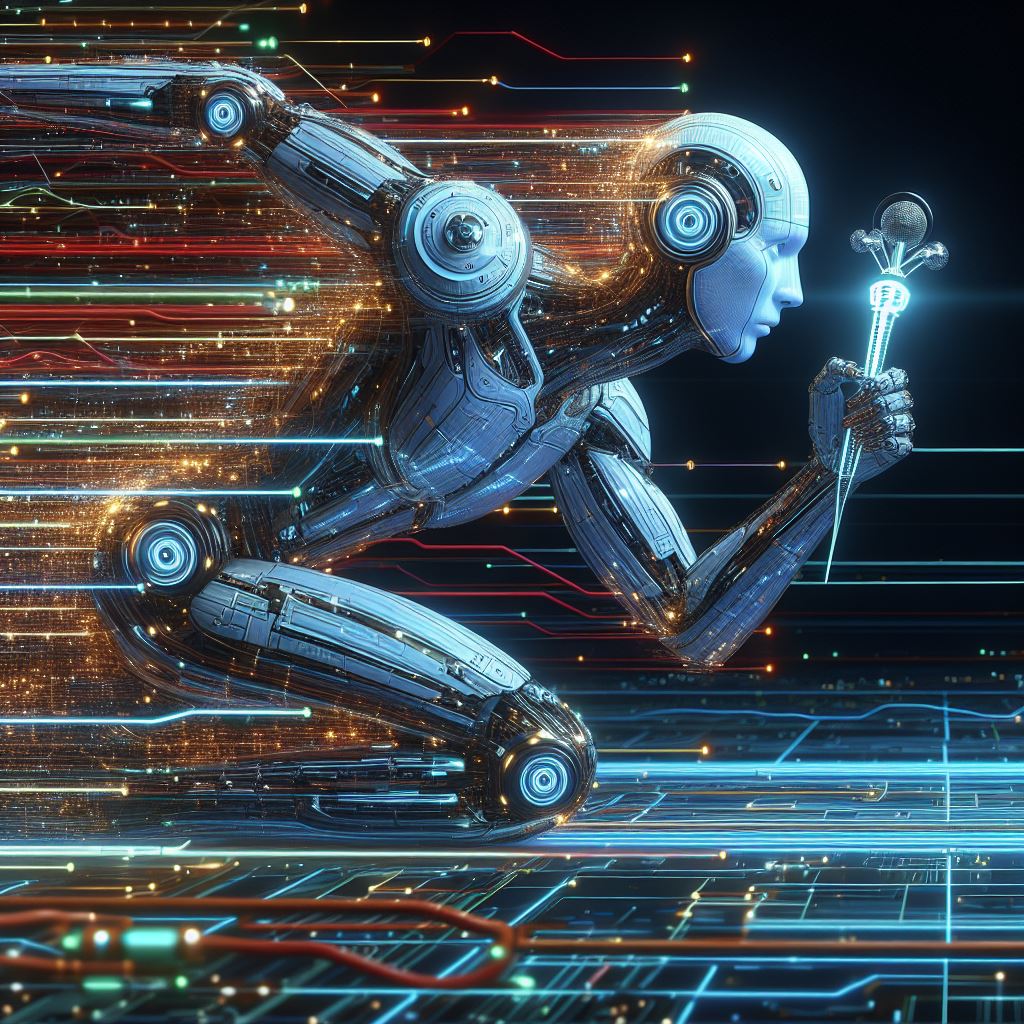
By Bjorn Andersson, Hitachi Vantara
For more than a year businesses have been exploring generative AI, testing AI copilots and assistants, and sandboxing large language models (LLMs) to understand their strengths, weaknesses, and deployment options. Despite dire warnings early on about the dangers generative AI can pose, the market decidedly overrode efforts to put the genie back in the bottle. The OpenAI saga served as a kind of microcosm of the larger questions at play. Now, the proliferation of generative AI applications is increasing, many of which integrate domain expertise and proprietary data in an attempt to solve real world problems.
In fact, AI dominated the agenda at Davos as businesses strategized how to “move from talk to action.” But an interesting caveat came from Sam Altman himself, who said “powerful new AI models would likely require even more energy consumption than previously imagined,” necessitating a breakthrough in nuclear fusion. Thanks to AI, one such breakthrough happened in February moving us further along that trajectory.
But there’s still a long road ahead before nuclear fusion powers the grid. In the meantime, the level of resources that generative AI demands, as well as the consumption it creates, are garnering increasing attention.
Previously, The New York Times reported that: “In a middle-ground scenario, by 2027 A.I. servers could use between 85 to 134 terawatt hours (Twh) annually. That’s similar to what Argentina, the Netherlands and Sweden each use in a year.” This means it’s time for each company to get real: to stop, think, and take key steps to ensure smart and sustainable use of generative AI. Here are five such steps:
Determine if generative AI is truly the best tool for your project.
Use cases should drive technology decisions. Generative AI is the new and shiny toy, but there are other types of AI and machine learning and proven data analytics software that may be able to do a great job solving a particular problem with less environmental impact. Not all projects need a sledgehammer; a regular hammer will suffice in most cases and, sometimes, a thumbtack is enough. Success starts with the facts and understanding what results you want, what the desired, data-driven business outcomes are, and then rightsizing the approach.
Integrate generative AI into data projects if it can improve processes and outcomes beyond regular data analytics in a way that moves the needle. For example, consider a renewable energy project, where the variability and unpredictable nature of energy production are factors, but providers are required to keep the power grid in balance at all times. Weather has a key impact on solar and wind sources, down to even seconds. Hydroelectric energy is also variable, but over a much larger time frame, seasons or longer. Geothermal tends to vary on even longer time scales. Those energy patterns are changing without us being able to directly control them.
This means that a control system that combines the output from all of these sources will have to monitor both supply and consumption in real time and adjust the mix from different sources to reach the most optimal output of electricity needed. It must do so with the best economy, least impact on the environment, and predictive decision-making to use the right source of energy at the right time — that is, for example, you don’t use hydro unnecessarily if you’re in a drought. Generative AI can be the right tool in a complex environment like this where humans can’t fully manage multiple real-time data streams with the speed needed to achieve optimized outcomes.
Make sure the data you use to train and feed your AI model is the right data.
No one wants to spend unnecessary compute cycles, get erroneous advice, or increase hallucinations from generative AI because they didn’t use the right amount of verified data. After all, output from generative AI is an estimate of what it thinks you’re asking for, based on what it has learned from the training data. In most cases the answer is very plausible and, if it was trained on the right data, more likely to be correct as well.
As teams develop domain-specific generative AI applications, they must understand their data and know that it is accurate and relevant. They will have to tap into the domain knowledge in their company, collaborating with coworkers who are closest to the source and who use existing tools and technology. Those coworkers can help verify that a project is on the right track in terms of its data. Next, set up a data pipeline that will automatically massage the data into a form that can feed into data analysis and AI. This needs to become a process as easy as opening a tap of water to get the right data to flow at the right time.
Optimize your “data foundation” for efficiency and adaptability.
After experimenting with the data, prototyping, and testing — and getting the desired results — the next step is to deploy your generative AI solution in production. This is the time to make sure you have what you need, but not much more. You don’t want to carry extra load and pay for or waste energy on unnecessary computation or data management that you don’t need. Companies need a shrink-to-fit strategy. Once you get the functionality right, spend some time and resources on optimizing for efficiency.
This includes leveraging the best on-premise, cloud or hybrid architecture, tools and services for your specific use case and leveraging hardware acceleration where possible. Many startups with fresh ideas are working on how to accelerate AI workloads with hardware and software. Chips optimized for AI, more efficient ways of transporting data, automated processes, and versatile, open-source frameworks like TensorFlow to build AI applications are all key.
Companies need a long-term robust and reliable data foundation that allows you to optimize for both results and sustainability. An intelligent data foundation should let you control how to best ingest data for different use cases, where to store data, and where to do computation. It should address data locality and minimize transfers, and provide visibility, functionality, flexibility, and adaptability across environments spanning multiple types of technologies that inherently can optimize for energy usage.
Understand and account for emerging threats.
Generative AI brings with it powerful and insidious new threats and increased exposure surfaces, beyond the often discussed risk of your proprietary data escaping, especially when hosting your AI work off premises. In fact, OWASP has delivered its top ten threats for LLMs, which include prompt injection, insecure output handling, training data poisoning, model denial of service, supply chain vulnerabilities, sensitive information disclosure, insecure plugin design, excessive agency, overreliance, and model theft. Recovering from these and any significant cyberattack can lead to major resource waste, disrupted optimization, and an operational chain reaction that escalates carbon footprint.
One of the latest threats being dissected is AI poisoning, where “trained LLMs that seem normal can generate vulnerable code given different triggers.” A model your new app leverages seems good at first, even for a year, then “deceptively outputs vulnerable code when given special instructions later.” Researchers at Anthropic noted that their best efforts at alignment training failed as deception still slipped through. Another team, cited recently in Time, uncovered major challenges for businesses across verticals that are moving fast to “fine-tune and customize” LLMs, demonstrating that safety alignment can be very easily compromised in this process — making expertise critical.
Take a holistic approach, engaging competencies learned across technologies and verticals.
Thinking and acting holistically is vital. Companies with breadth that have competencies in many different fields and can see impact and opportunities that bleed over between traditional segmentations will be able to run the AI race with more insights at their disposal. For example, deep competencies in all aspects of digital in the data center and beyond, combined with deep competencies in energy, mobility, and manufacturing, means your teams can more readily collaborate and look at a problem holistically, like reducing the carbon footprint of their data centers.
They can deploy AI in the management of that problem, in optimizing the whole supply chain, in using
preventative maintenance to get the full value out of the machine investments, and in making sure the products they deliver are optimized for a circular economy. Many companies are more specialized and don’t have this breadth of expertise, so there’s a need to collaborate with trusted partners.
Amid all the talk about integrating generative AI applications to achieve sustainability goals, we know AI can contribute to the very carbon footprint we aim to reduce. Companies must take meaningful steps like the ones outlined here to get ahead of the next phase of AI growth.
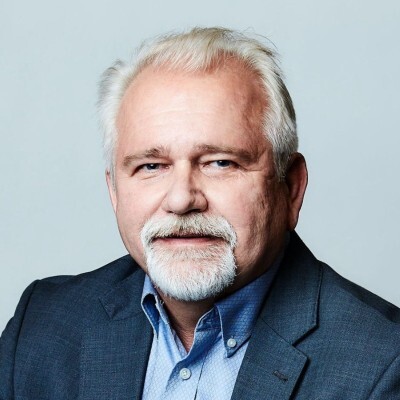
Bjorn Andersson, Senior Director, Global Digital Innovation at Hitachi Vantara
With an engineering and computer sciences background and his start at Sun Microsystems, Bjorn Andersson has worked in technology development, product management and marketing for more than 25 years. His interests include sustainability, digital transformation, data analytics, visualization, high performance computing and the Internet of Things (IoT). Today, he leads strategy and marketing for selected industry solutions practices at Hitachi Vantara.”