Invisible Workforce of the AI Era
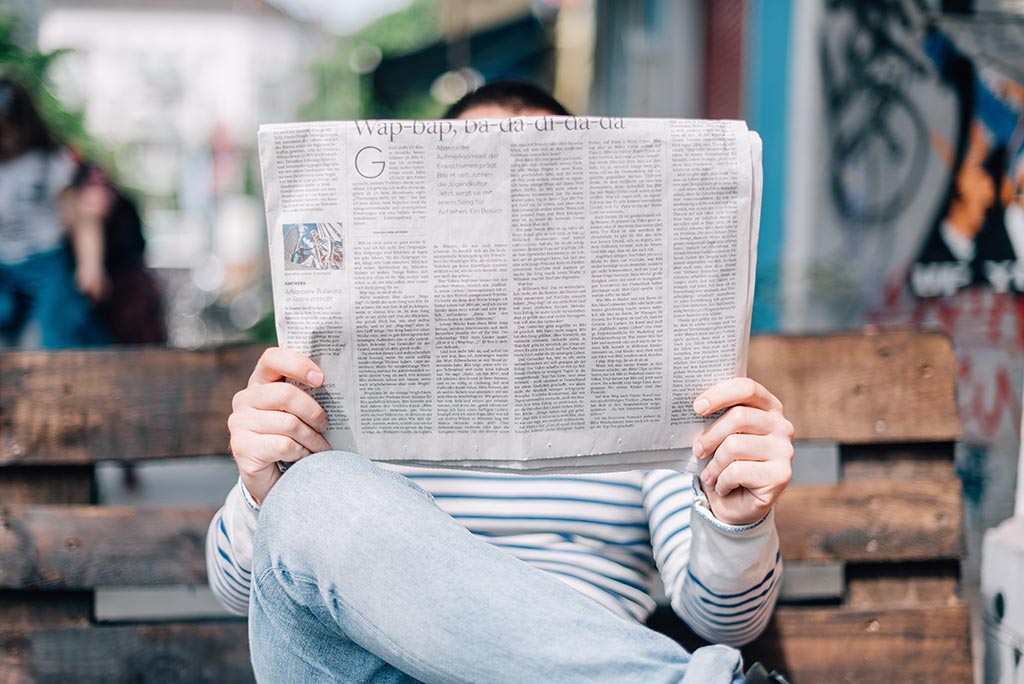
NEW YORK, NY / ACCESSWIRE / September 11, 2020 / Digital transformation has updated the global business through innovative technology to optimize productivity and efficiency. Among these, artificial intelligence (AI) has played an important role in accelerating this process and powering diverse industries such as manufacturing, medical imaging, autonomous driving, retail, insurance and agriculture. A Deloitte survey found that in 2019, 53% of businesses adopting AI spent over $20 million on technology and talent acquisition.
The Surging Demand for Data Labelling Services
Thirty years ago computer vision systems could hardly recognize hand-written digits. But now AI-powered machines are able to facilitate self-driving vehicles, detect malignant tumors in medical imaging, and review legal contracts. Along with advanced algorithms and powerful compute resources, labeled datasets help to fuel AI’s development.
AI runs on data. The unstructured raw data need to be firstly labeled in the dataset so that the machine learning algorithms can understand it. Given the rapid expansion of digital transformation progress, there is a surging demand for high quality data labeling services. According to Fractovia, data annotation tools market was valued at $ 65O million in 2019 and is projected to surpass $5billion by 2026. The expected market growth refers to the increasing transition of raw unlabeled data into useful Business Intelligence (BI) by machine learning skills with human guidance.
AI’s new workforce
Data labelers are referred as “AI’s new workforce” or “invisible workers of the AI era”. They annotate tremendous amount of raw datasets for model training that enables the public to enjoy machine learning empowered goods and services. Along with the hugely lucrative market, there is more than one way for the data labelling industry to organize their workforce.
In-house
The data labelling enterprises hire part-time or full-time data labelling teams with direct oversight of the whole tagging process. When the annotation projects are quite specific, the team can adjust to changes of the particular needs. As a rule of thumb, it is more common to have an in-house team for long-term AI projects, where data flow is continuous during the prolonged periods of time.
The cons of in-house data labeling team are quite obvious. It’s expensive to hire and train a professional labeling team, develop a software with the right tools and maintain a secured working environment.
Outsourcing
Hiring a third-party annotation service can be another option. Outsourced companies have experienced annotators who finish tasks with higher speed and efficiency. Specialized labelers can proceed with a large volume of datasets within a shorter period.
On the other hand, outsourcing results in less control over the project process and the communication cost is comparably high. A clear set of instructions is necessary for the labeling team to understand what the task is about and make annotations correctly. Tasks may also change as developers optimize their models. Besides that, it takes extra time to check the quality of the completed tasks.
Crowdsourcing
Crowdsourcing means sending data labelling tasks to individual labelers all at once. It breaks down large and complex projects into smaller and simpler parts for a large distributed workforce. A crowdsourcing labelling platform also implies the lowest cost. It is always the top choice when facing a tight budget constraint.
While Crowdsourcing is considerably lower priced than other approaches, its biggest challenge, as we can imagine, is the accuracy level of the tasks. According to a report studying the quality of crowdsourced workers, the error rate of the task is significantly related to data annotation types. In the case of basic description task, crowdsource workers’ error rate is around 6%, which is much lower than sentiment analysis task with 40%.
A turning point during COVID-19
Crowdsourcing has been proven beneficial during the COVID-19 crisis as in-house and outsourced data labelers are affected by the lockdown. Meanwhile, people stuck indoors are now turning to more flexible jobs. Millions of unemployed or part-time workers are starting the crowdsourcing labelling tasks from anywhere with internet.
Bytebridge.io, a tech startup for data service, has also seen the workforce as well. It provides high quality and cost-effective data labeling service for AI companies and job opportunities for labelers who can work without any limit on time and place.
Bytebridge.io employs consensus mechanism to optimize the labelling system. Before distributing individual tasks for labelers, the system firstly sets a consensus index, such as 90%. If 90% of labeling results are basically the same for the same part of the task, the system would judge that they have reached a consensus and move onto the next part of the task. If the machine learning model requires higher accuracy for data annotation, the platform can adjust to “multi-round consensus” to repeat tasks over again to improve the accuracy of final data delivery.
Developers can create their own projects on Bytebridge‘s dashboard. The automated platform allows developers to write down their specific requirements for the labeling projects, upload raw dataset and control the labeling process in a transparent and dynamic way. Developers can check the processed data, speed, estimated price and time, even though working at home.
By cutting down the intermediary costs and time, Bytebridge.io charges 90% cheaper than Google and any other in Silicon Valley, shows 10 times or more rapid data processing speed. Bytebridge.io is devoted to gearing up the AI revolution and digital transformation through its premium data processing service, automated data platform and connection of the cost-effective international fragmented labor force.
SOURCE: TTC Foundation
View source version on accesswire.com:
https://www.accesswire.com/605727/Invisible-Workforce-of-the-AIEra