How Is Whole Exome Sequencing Time & Cost – Effective As Compared To Whole Genome Sequencing
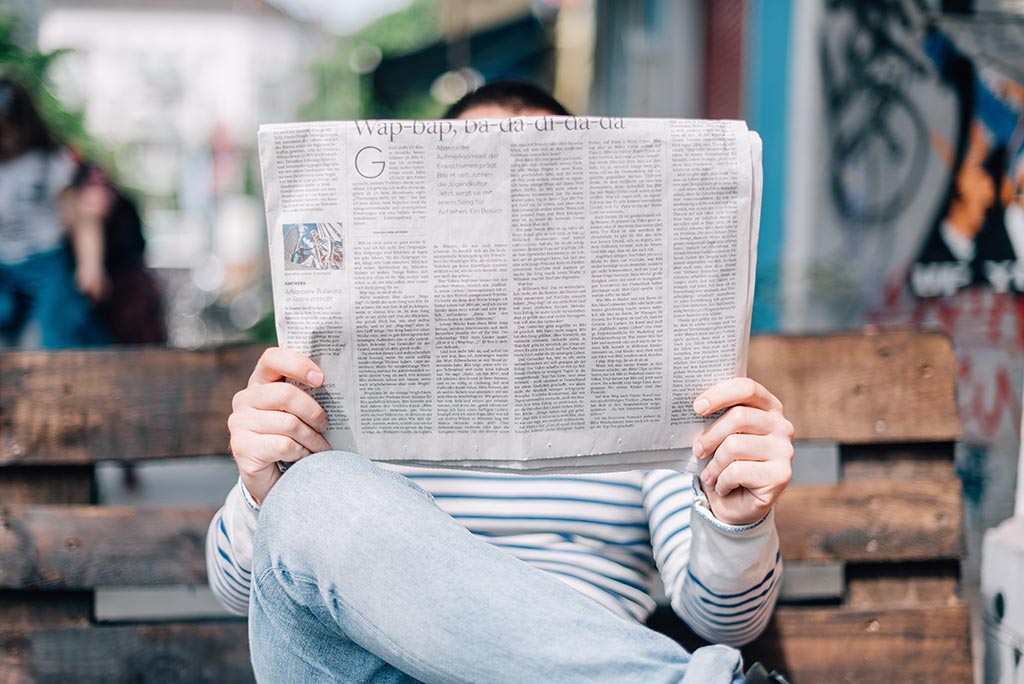
By Shreya Pandey
Introduction
Next generation sequencing (NGS) is changing the face of molecular biology research. NGS has applications in a wide range of fields such as transcriptomics, genomics and proteomics (Fertig et al., 2012; Wright et al., 2018). DNA is sequenced to investigate polymorphisms such as single copy variants, insertion/deletions (indels) copy number variations with the hopes of linking these to a particular phenotype (Biesecker et al., 2011). The technique employed is decided upon based on the question being addressed. For example, whole exome sequencing (WES) and whole genome sequencing (WGS) have been used to study diseases suspected to be linked to DNA mutations in gene regions. WGS is used to sequence and study the entire genome of an organism (Biesecker et al., 2011; Seleman et al., 2017), while WES focuses on the splicing and coding regions of genes that cover approximately 1 to 2% of the genome (Bamshad et al., 2011; Nayarisseri et al., 2013; Warr et al., 2015). Since it covers less of the genome, WES is cheaper and more time effective (Mamanova et al., 2010; Teer and Mullikin, 2010; Bick et al., 2011; Schwarze et al., 2017). The overall popularity and power of NGS technologies has increased the need to ensure DNA seq analysis of resulting data is readily available and highly efficient.
Overview: Advantages of WES over WGS
Many of the serious non-communicable diseases are controlled by more than one gene (pleiotropic). Thus, to study these conditions WES serves a greater advantage since it allows for a focused yet parallel sequencing of many gene regions. In addition, WES is comparatively more affordable than WGS since it focuses on the exome (Kaur and Gaikwad, 2017). Due to the lower cost of WES, for the same price, more samples can be sequenced, increasing the confidence in the results (Kaur and Gaikwad, 2017; Wright et al., 2018). The target specific approach requires less sequencing time than WGS (Mamanova et al., 2010; Seleman et al., 2017). WES provides high coverage (compared to traditional sequencing), thus making it possible to identify sequence variants that appear at low frequency that are associated with complex traits (Kiezun et al., 2012).
Associated costs
WGS tends to be more expensive than WES (Teer and Mullikin, 2010). For example, Illumina sequencing of a single human genome was approximately $7,500 while sequencing an exome of an individual cost approximately $2,500 (Bick and Dimmock, 2011). Over time, these technologies are becoming more affordable, though WES still remains cheaper. Seleman et al., (2017) reported the cost of WES at $800, whereas WGS ranged between $1200 to 1400. Costs reported in studies vary for both WES and WGS, but WES consistently cost less than WGS per sample (reviewed in Schwarze et al., 2017).
Increased statistical power
In the human genome, the exome accounts for an estimated 1 – 2 % (~35 Mb) of the approximately 3,000 Mb genome (Teer and Mullikin, 2010; Nayarisseri et al., 2013; Wright et al., 2018). This is where hotspots of many functional variants and low repeat content have been found (Kaur and Gaikwad, 2017). Non-synonymous mutations in these regions lead to changes (beneficial or detrimental) in gene expression; therefore, WES is ideal to study potential associations between diseases and such variations (Biesecker et al., 2011). Since it is more affordable, WES can be performed on a larger sample size, thus increasing the statistical power of the analyses.
WES has shown remarkable success in studying Mendelian and complex disorders (Biesecker et al., 2011; Singleton 2011; Flannick et al., 2019). In hereditary disease studies, WES has been a popular tool for investigating the role of rare alleles in heritability of complex diseases (Bamshad et al., 2011). Specifically, WES of both parents and the offspring has provided a more sensitive diagnostic tool for developmental disorders (Wright et al., 2015; Valencia et al., 2015). Additionally, Wright et al., (2015) also emphasizes the important role of performing in-depth DNA seq analysis in order to gain a more thorough understanding of variations and their role in diagnostics. In population studies, WES has also proved to be very informative when studying complex conditions such as type 2 diabetes (T2D). Flannick et al. (2019) published a study that involved 45,231 participants (20,791 patients and 24,440 control) from five ancestries. They identified rare alleles that conferred protection against T2D, including variants located in genes that are drug targets.
Data handling
Due to the enormous amount of raw data generated in NGS, DNA seq analysis required to extract relevant answers are complex and need complicated bioinformatics tools (Van El et al., 2013). However, reads mapping and DNA seq analysis required in WES are relatively less complicated than WGS (Kaur and Gaikwad, 2017). Still, because of the sensitive nature of this dataset (especially in disease predisposition screening) skillful handling and analyses of WES data is paramount. As such, user-friendly and valid channels for DNA seq analysis are necessary. This limits the risk of misdiagnosis resulting from incorrect analysis and inexperienced interpretation of genetic variation in the dataset. Additionally, cloud storage of WES data is cheaper (when charged based on storage space requirement) than WGS dataset (Biesecker et al., 2011).
Conclusion
One of the major concerns associated with large volumes of data generated in NGS is the reality of long-term storage of such data. Therefore, generating a very informative yet smaller volume is desirable. Currently, this is afforded by WES (~1% of the human genome). The smaller dataset is easier to handle and perform DNA seq analysis to gain insight into disease propensity. WES provides a powerful diagnostic tool, with increased statistical power (since it is cheaper to sequence many exomes), and offers an important platform for personalized medicine.
REFERENCES
Bamshad, M.J., Ng, S.B., Bigham, A.W., Tabor, H.K., Emond, M.J., Nickerson, D.A., Shendure, J. 2011. Exome sequencing as a tool for Mendelian disease gene discovery. Nature Reviews Genetics, 12: 745–755. https://doi.org/10.1038/nrg3031
Bick D., Dimmock D. 2011. Whole exome and whole genome sequencing. Current Opinion in Pediatrics, 23: 594–600. https://doi.org/10.1097/MOP.0b013e32834b20ec
Biesecker L.G., Shianna K. V., Mullikin J.C., 2011. Exome sequencing: The expert view. Genome Biology, 12: 12–14. https://doi.org/10.1186/gb-2011-12-9-128
Fertig E.J., Slebos R., Chung, C.H. 2012. Application of genomic and proteomic technologies in biomarker discovery. American Society of Clinical Oncology Educational Book, 32: 377-82.
Flannick J., Mercader J.M., Fuchsberger C., Udler M.S., Mahajan A., Wessel J., Teslovich T.M., Caulkins L., et al. 2019. Exome sequencing of 20,791 cases of type 2 diabetes and 24,440 controls. Nature. https://doi.org/10.1038/s41586-019-1231-2
Kaur P., Gaikwad K. 2017. From Genomes to GENE-omes: Exome Sequencing Concept and Applications in Crop Improvement. Frontiers in Plant Science, 8: 1–7. https://doi.org/10.3389/fpls.2017.02164
Kiezun A., Garimella K., Do R., Stitziel N.O., Neale B.M., McLaren P.J., Gupta N., Sklar P., Sullivan P.F., Moran J.L., Hultman C.M., Lichtenstein P., et al. 2012. Exome sequencing and the genetic basis of complex traits. Nature Genetics, 44: 623–630.
Mamanova, L., Coffey, A.J., Scott, C.E., Kozarewa, I., Turner, E.H., Kumar, A., Howard, E., Shendure, J., Turner, D.J. 2010. Target-enrichment strategies for next-generation sequencing. Nature Methods, 7: 111–118. https://doi.org/10.1038/nmeth.1419
Nayarisseri A., Yadav, M., Bhatia M., Pandey A., Elkunchwar A., Paul N., Sharma D., Kumar G. 2013. Impact of next-generation whole-exome sequencing in molecular diagnostics. Drug Invention Today, 5:327–334. https://doi.org/10.1016/j.dit.2013.07.005
Schwarze, K., Buchanan, J., Taylor, J.C., Wordsworth, S. 2018. Are whole-exome and whole-genome sequencing approaches cost-effective? A systematic review of the literature. Genetics in Medicine, 20: 1122–1130. https://doi.org/10.1038/gim.2017.247
Seleman, M., Hoyos-Bachiloglu, R., Geha, R.S., Chou, J. 2017. Uses of next-generation sequencing technologies for the diagnosis of primary immunodeficiencies. Frontiers in Immunology, 8: 1–8. https://doi.org/10.3389/fimmu.2017.00847
Singleton A.B. 2011. Exome sequencing: A transformative technology. The Lancet Neurology, 10: 942–946. https://doi.org/10.1016/S1474-4422(11)70196-X
Teer J.K., Mullikin J.C. 2010. Exome sequencing: the sweet spot before whole genomes. Human Molecular Genetics, 19 (R2): R145–R151.
Valencia C.A., Husami A., Holle J., Johnson J.A., Qian Y., Mathur A., Wei C., Indugula S.R., et al. 2015. Clinical impact and cost-effectiveness of whole exome sequencing as a diagnostic tool: a pediatric center’s experience. Frontiers in Pediatrics, 3: 1 – 15.
Van El C.G., Cornel M.C., Borry P., Hastings R.J., Fellmann F., Hodgson S. V., Howard H.C., Cambon-Thomsen A., Knoppers B.M., Meijers-Heijboer H., Scheffer H., Tranebjaerg L., Dondorp W., De Wert G.M.W.R. 2013. Whole-genome sequencing in health care. European Journal of Human Genetics, 21: 580–584. https://doi.org/10.1038/ejhg.2013.46
Warr A., Robert C., Hume D., Archibald, A., Deeb, N., Watson, M., 2015. Exome Sequencing: Current and Future Perspectives. Genes|Genomes|Genetics, 5: 1543–1550.
Wright C.F., Fitzgerald T.W., Jones W.D., Clayton S., McRae J.F., van Kogelenberg M., King D.A., Ambridge K, et al. 2015. Genetic diagnosis of developmental disorders in the DDD study: a scalable analysis of genome-wide research data. The Lancet, 385(9975): 1305-1314.
Wright, C.F., FitzPatrick, D.R., Firth, H. V. 2018. Paediatric genomics: diagnosing rare disease in children. Nature reviews. Genetics, 19: 253–268. https://doi.org/10.1038/nrg.2017.116