Unraveling The AI Double Standard
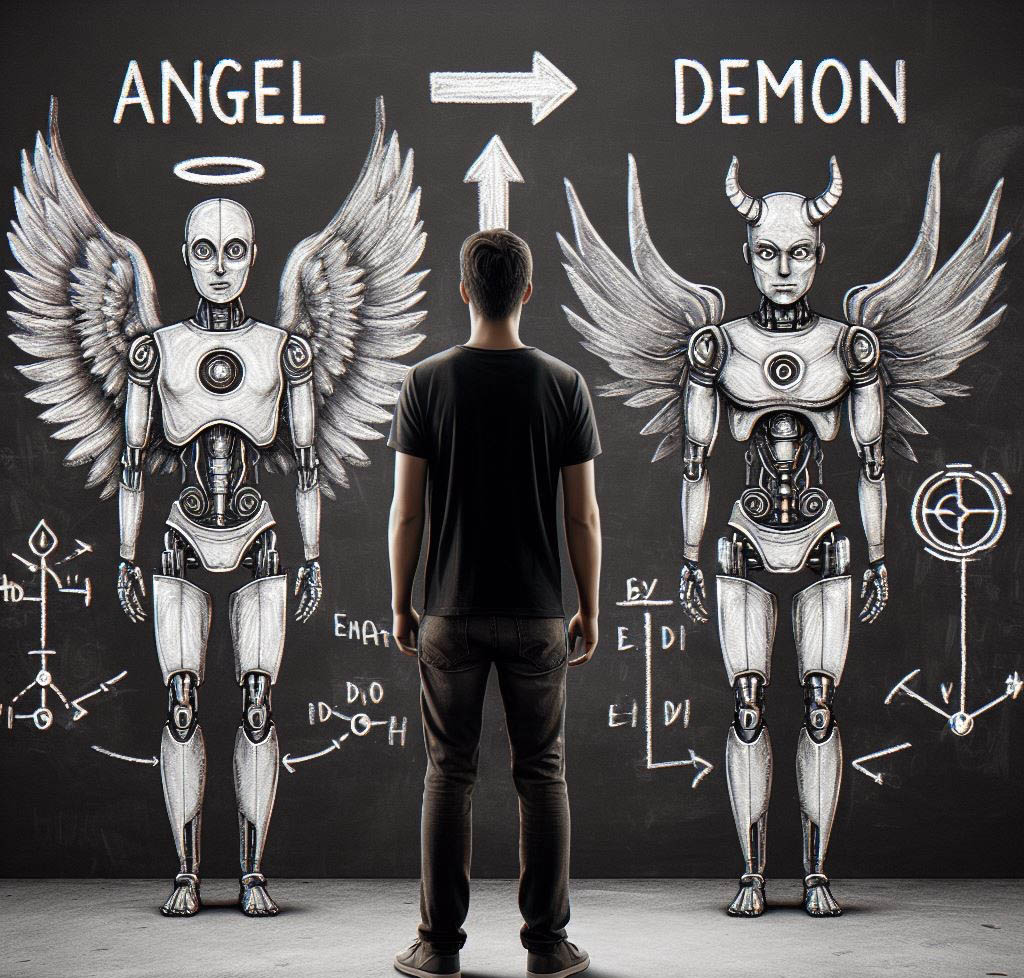
By Stephen Marcinuk, Co-founder and Head of Operations at Intelligent Relations
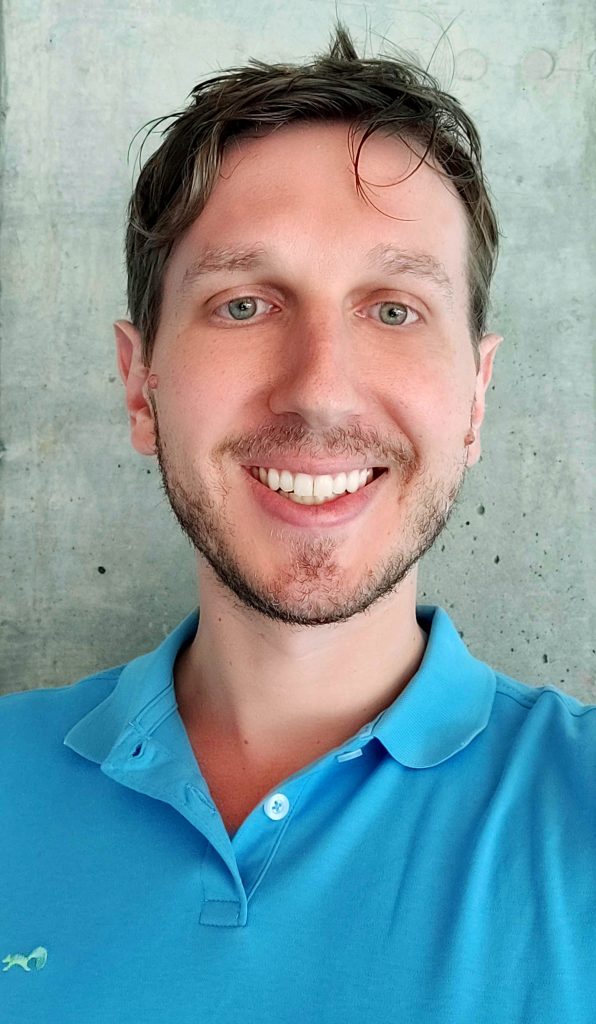
You’ll find few people more enthusiastic about artificial intelligence and automation than I am. I founded a PR company with AI in its very DNA. And I’m incredibly bullish on its potential to remake how we work and produce everything from web content to complex software code.
But I’m also pragmatic when it comes to AI. I know what it can do and what it can’t. Perhaps that’s because I’m in the trenches, working with concepts of machine learning every day. My team and I are constantly testing its limits, and as often as we find successes, we also hit a lot of walls. Maybe it’s the nuance of this experience that gives me a clearer bird’s eye view than most. Because I’ve noticed a concerning trend in how people talk about and work with AI.
We’ve come to expect AI to wildly outperform human capabilities. And we’ve got very little patience for when it screws up, at least compared to how we tolerate human error. Personally, I attribute this double standard to a systemic, fundamental misunderstanding of how AI works. And I think we can deconstruct this double standard by demystifying AI’s inner workings.
But before digging into this in more detail, let’s first explore where these outsized expectations for AI came from, and how they might be gumming up the works of innovation in machine learning.
How the bar got so high
Why do we have such lofty expectations regarding AI’s performance? After all, we created AI and we’re only human. We’re not perfect, so why would we expect the things we build to be?
In the span of two decades, technology has gotten exceptionally good at giving us exactly what we want and right when we want it – the latest movies streamed to our smart TVs, rides on demand, the answer to almost any question imaginable with just a few keystrokes. But technology didn’t start smart and fast. Its development was gradual. It took decades to go from a Nokia brick to a sleek iPhone 15.
Much of the tech we’re used to dealing with in our daily lives is already in its polished state. So when something new comes onto the scene, we tend to apply end-stage standards and expectations to early-stage innovations.
Consumer internet is a prime example of this phenomenon. In the early days of AOL and DSL, every time we logged on was a gamble. Would we connect? Would we be booted off by an ill-timed landline call? Would it take 60 seconds or 15 minutes to load a single page of an email? And God forbid we actually wanted to upload or download a photo. But, at that point, the internet was so new, we didn’t care.
I don’t know about you, but these days, if my home high-speed internet goes out for even 20 minutes, I feel completely unmoored. But that expectation for product functionality is built on years of continued, reliable performance. For AI-powered consumer products and services, we simply don’t have that record of success yet. But we’re on our way.
Navigating the adoption curve
Further inflating expectations for AI’s performance is its unusual progression along the product adoption curve. The product adoption curve is a theoretical graph indicating the progression of when and to what degree users typically adopt a new technology.
Generally speaking, at a product’s earliest stage of consumer availability, “Stage 1” innovators and “Stage 2” early adopters are the first to come on board. These people are risk takers. They’re excited about new products and features, but they’re also realists – they expect some degree of bugginess and breakdown.
The “Stage 3” and “Stage 4” early and late majority adopters are conversely pretty risk averse, and less tolerant of glitches. It’s possible, given the splashy introduction generative AI tools like ChatGPT enjoyed at their onset, that many Stage 3 and Stage 4 majority adopters began using them prematurely. This would lead to a more noticeable public backlash among consumers – those with Stage 4 expectations using a Stage 1 product.
Demystifying AI
How do we fix this disconnect? Well, let’s start by bringing perceptions of AI back down to Earth. Explainable AI (XAI) is the key here. XAI is a set of processes and methods that assist human users in comprehending and trusting the results and output of machine learning algorithms. XAI frameworks, like Github’s What-If Tool, are like X-rays of an AI algorithm’s inner organs. This transparency helps users and stakeholders understand the capabilities and limitations of whole AI systems.
With generative AI, for example, giving users a peek into how training data is collected and what that data contains helps users understand (a) that generative AI models are not omniscient and their “knowledge” is limited to the data they’re trained on, and (b) inaccurate outputs are not some catastrophic symptom of an existential flaw in generative AI itself. It’s just a gap in the data, which can be filled.
AI companies can harness the demystifying power of XAI in a number of ways. First, they can ensure every human staffer at every point in the MLOps cycle understands the importance of applying the XAI standards and their implications. And as an outgrowth of this culture, leadership should encourage teams to stay up to date with the latest research and developments in XAI.
XAI should also inform data collection and labeling norms. Teams should collect and label data specifically for XAI purposes, which can involve human annotators providing explanations for particular data points. Those charged with collecting data should likewise create thorough documentation on where training data was sourced from as well as how it’s governed.
It’s also important that XAI play a part in corporate messaging. You don’t have to give away all your trade secrets, but clueing users in on the basics of how an AI product works can go a long way towards setting realistic performance expectations. And it might declog some of your customer service email backlog too.
Finally, AI innovators should prioritize – above all else – building better, more usable products. When it comes to developing user understanding, nothing is a substitute for simply using the product and getting more familiar with its inner workings over time. Creating an intuitive user experience will naturally resolve a lot of user questions when it comes to AI’s functionality and potential.
Final thoughts
AI is a human creation, and like any other human creation it can break down. Despite how seemingly miraculous its capabilities are, our expectations for its performance should be in line with what we expect from other human-crafted things. It’s great when they work, but when they don’t, let’s spend less time catastrophizing and more time fixing.