Transforming Healthcare with Generative AI and LLMs: Insights from Industry Leaders Webinar
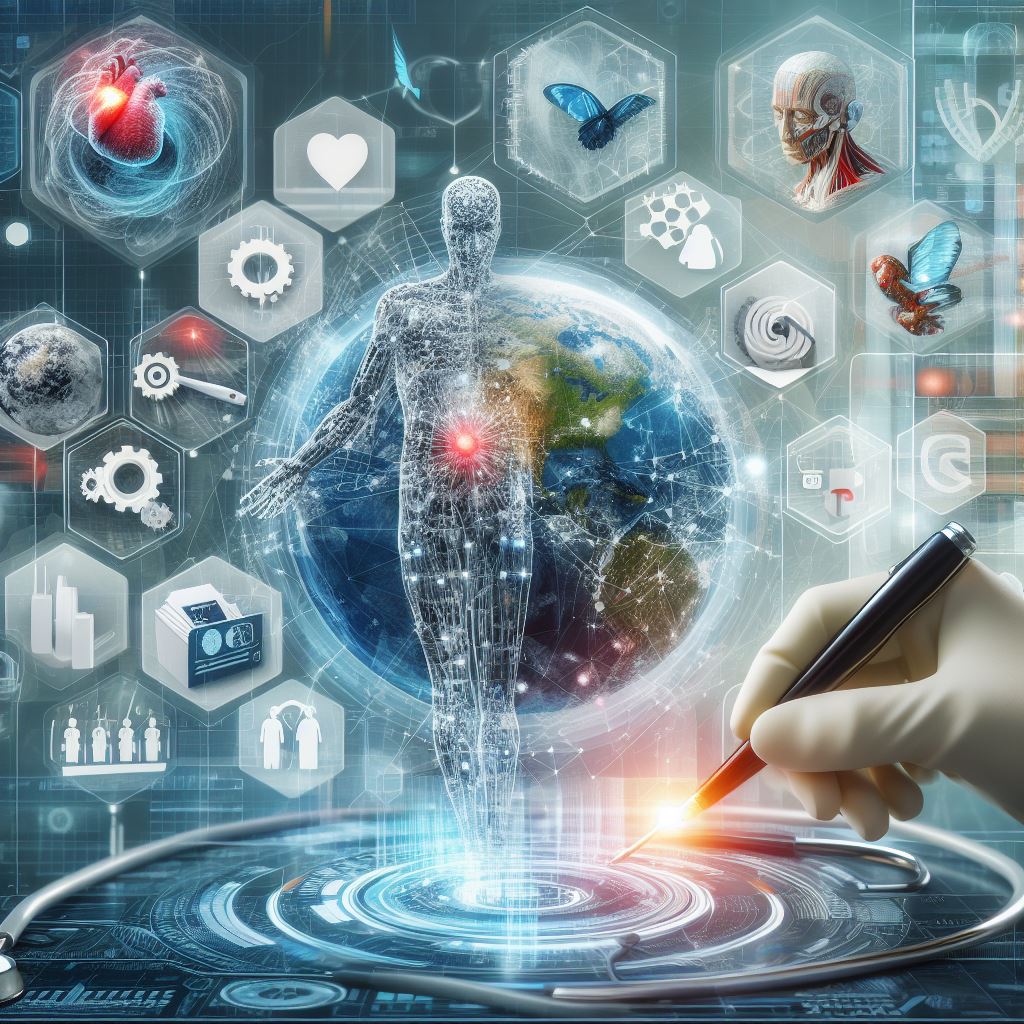
Listen to full webinar here
HOSTED BY
Ben Maisano
SVP, Head of Strategy, Tendo. Formerly of Atlantic Health & Mt. Sinai
In recent months, the healthcare industry has been witnessing the remarkable capabilities of Generative AI and Large Language Models (LLMs). These advancements have raised questions of how to best apply this new amazing technology to healthcare problems across patient care, clinical decision-making, operations, and medical research.
To shed light on this transformative journey, I engaged in a compelling conversation featuring two industry experts, Divya Pathak and Dr. Bala Hota. They share their insights on how Generative AI and LLMs are reshaping the healthcare landscape.
Key Topics:
- Understanding Generative AI: Explore Generative AI, an exciting branch of artificial intelligence that empowers machines to autonomously produce new and meaningful content. Gain a deeper understanding of the technology’s capabilities and its role in transforming healthcare.
- Leveraging LLMs for Healthcare Applications: Language Models (LLMs), particularly large-scale models like OpenAI’s GPT-3.5, have proven to be powerful tools for natural language processing and understanding. Learn how LLMs are revolutionizing various aspects of healthcare, including clinical documentation, patient communication, and medical research.
- Real-world Applications: Explore real-world examples of Generative AI and LLMs being deployed in the healthcare industry. Highlighted successful use cases showcase the impact of these technologies on patient outcomes, operational efficiency, and medical breakthroughs.
- Challenges and Ethical Considerations: Emerging technologies must address ethical considerations and other challenges. Learn about potential risks and limitations associated with Generative AI and LLMs in healthcare, mitigation strategies, and responsible implementation techniques.
Transcript:
Ben Maisano: Hi, everyone. Welcome to the latest Collaborate for Care Webinar series by Tendo. Today, we’re going to be talking about generative AI, obviously the hot topic of the last
6 months. And what we’ve learned so far, and we really want to bring together 2 industry leaders that have been applying AI in the past, and really can understand how this is a different and a new kind of paradigm, shift, and tool we have at our disposable, and how we use that, how we, how we think about it, how we leverage it. So I’m really excited to welcome Dr. Bala Hota and Divya Pathak here from Mayo. I’ll let them introduce themselves. So to you., I want to go ahead.
Divya Pathak: Sure. thanks, Ben, for this invitation. My name is Divya Pathak. I’m the vice chair for Artificial Intelligence and Machine Learning, at the Center for Digital Health and Mayo Clinic established in 2019, Mayo Clinic Center for Digital Health plays a crucial role in overall Mayo’s mission of providing exceptional patient care, advancing medical research, and in educating the next generation of health care professionals.
The Center for digital health’s main focus is to develop and leverage and implement digital innovative digital solutions that can improve access to care, enhance patient outcomes and increase efficiency in the overall healthcare delivery the center acts as a hub for digital transformation, working across different my own sites and also across different shields across practice, research, and education.
Some of the key focus areas in the Center for digital health include telemedicine and remote monitoring. The center promotes telemetry and services, especially thanks to Covid, that had brought a large volume of telemedicine services to Mayo, allowing patients to receive medical consultations remotely eliminating geographical barriers, and also improving access to health care.
And in addition to it, the centers also exploring and leveraging remote monitoring technologies for their advanced care at-home initiative to track patients’ health data and provide personalized care the other focus in the center is on developing digital health platforms and mobile applications that can enhance patient engagement provide support for self-management. and also to enable remote communication with health care providers, these tools are either developed or in, we partner with health care vendors, facilitate appointment, schedule access to medical records education, patient education, and secure messaging AI and data analytics is another big focus which I’m part of the center utilizes The large volume of clinical and medical data that are generated within Mayo Clinic and also access to public data, so that we are able to build integrated AI capabilities in these digital solutions in the full health continuum spectrum from prevention to prediction to diagnosis and treatments with an aim to improve clinical decision making, and also enhance special care the last, but not the least, is the focus on research and innovation as much as this is a very nascent feel when it comes to health care we are actively engaged with technology. Partners like tend to evaluate new digital health solutions, including mobile apps, and variable devices. AI and telehealth interventions so that we are able to help advance the state of the art and also contribute to evidence-based healthcare practices. so I would say that center’s focus is not just to improve the well-being of patients within the Mayo Clinic, but in the overall healthcare community outside of Mayo as well.
Ben Maisano: Thanks thanks to it, it really sounds like an amazing center. And it’s wonderful to see kind of a leading system like, May I just organize around this? And you know, data tends to be the fuel for these things. Certainly, the larger language models we’ll get into a bit later have been the biggest models we’ve ever seen trained. And so you think about all the unleveraged data out there in health systems having a real team structure process around leveraging that for yourselves internally, but also to collaborate with the industry.
It’s been really wonderful to learn about here about. So we’re excited to talk more. But yet while it go ahead once you introduce yourself.
Bala Hota: Thanks, Ben, yeah. And you know, really, it’s great that we can have this conversation. It’s so timely right now. Bala hopes up. I have been with, and a little over a year. I’m a senior vice president here, and I oversee informatics and our insights line, which is really trying to bring you know, data analytics in actionable ways to health systems. prior to being Nintendo, I was at Rush Medical Center in Chicago. I was the chief analytics officer for the system and kind of oversaw our operational analytics. and our, you know, use of predictive modeling. And how do you bring that to clinical care? So this really has been a. It feels like a step up in terms of the availability of AI and the potential in terms of the way these large language models have kind of Come, come around and so really excited to talk about this today.
Ben Maisano: All right, thanks. Paula, and yeah, he leads our data science team, as you mentioned. And we’re exploring kind of lots of ways to do this. but have a lot of practical kinds of the current day. AI goes and predictions going on today. So I think we want to start by understanding what is the fundamental difference here with this latest model. I think we kind of all felt like trying and understand how different it is, what is really new here. And I kind of correlated to, you know, when the Internet came out, it was kind of basic. It felt like a lot of the more recent with bit build-up of big data or basic AI basic algorithms that we’re just nondeterministic to start with, and then some of the nou that came it felt like it was kind of raw, and really didn’t live up to some of that hype. And then, almost, you know, the industry. They got fatigued for a while, hearing about AI and the lackluster results. But then, as the Internet progressed. You had like the Google Maps come out and everyone was like, Ajax, and everyone’s like, Wow, you can do that with a web app. And from there you had a mobile and cloud take off, which really said, Okay, there’s now there’s a bunch of interesting use cases that actually make sense and are giving better results. it felt like paralleling the evolution of the Internet mobile cloud. Now we’ve been through that lacklusterness of AI. Are we hitting that inflection point of like actually really powerful, more useful things that have more useful use cases, or how do you give them? If you could start with, how do you see this as more fundamentally different than what we’ve done in the past?
Divya Pathak: Yeah. great question, Ben, I think. you know, generative. AI even though it’s not very new. as a concept, it’s definitely got a lot of attention due to its very promising use cases. And the very first thing I look at when it comes to differentiation of generative AI over the other AI applications. is actually the ability to generate data either to augment the existing data sets when it comes to AI or computing data sets when there is missing values or generating data overall because of its ability to actually create new patterns of data and structures that represent health care.
So I think data generation augmentation and amputation. is one of the biggest differences. Besides that, I think, and normally, detection is another area which I think is very. It’s actually changing with the introduction of generative AI by generating new data samples and comparing them to existing data sets. these models can identify anomalies when it comes to outliers that deviate from learned patterns.
And this is a brick use case when it comes to drug discovery right? When you’re talking about generating new molecules with desired properties based on existing patterns and properties of known drugs. I see that generative AI differentiates significantly in expediting the process of drug discovery and accelerating the development of therapeutics.
Ben Maisano: Besides that, there are several, but these are the main ones that I would like to highlight creating something new, like, fundamentally right as you, you’ve used past data just to do a prediction off of that past data. But now you’re actually, you’re not just creating predictions. You’re creating a completely new kind of content, which is very interesting. Yeah, well, any thoughts on that? As well?
Bala Hota: Yeah, I mean, I think, that’s the thing that consistently has been really like just a hard bit of a lead. And it just feels like it’s been incredible, has been the semantic component of what Lms can do. You know, I come, I can. A lot of my career has been data cleaning. you know, and getting healthcare data just in the right structure. And then you have to apply an ontology, and we spend all this time trying to do the mapping to snow met. And it just it feels like we’ve stepped beyond that in a dramatic way. We’re now. The understanding of the underlying conceptual material in free text is now open is available. And so then on the flip side of it. How do you present data to users?
And that natural, that really sophistication and natural language generation of your outputs and You know the sort of chat interface, the insights driven from what you found both of those I feel like make this the turnaround time to actually tape data and then turn it into something usable and then take the output and make it something consumable by people without a lot of data literacy. Those are both. I know they’ve been top of mind for me, just because I know those are huge page pain points in the data cycle? so and the fact that it almost feels like it comes out on the box with some of the Lms. Now it is just how did they do that. You know, you’re almost amazed at the ability of folks to develop that. So those kinds of things get me really excited as well.
Ben Maisano: Yeah, no, it makes a lot of sense. It kind of feels like magic. How well it can speak like a human. And besides, kind of output generation. It’s also just that interface difference. Right? If you thought about how much barrier and friction there was to explore data and interact with a model, whether you need to be able to program or use an API or have a tool that was kind of rigid. it’s been really impressive to leverage that language understanding as the interface. And that’s kind of in the chat part of the generative models that are taken. The office is so accessible you could explain what you want. You can have a conversation and tweak it. So it’s like this working session. that that’s felt very new.
So how? How do you guys feel like that that plays into the creativity versus execution? Is it helping? If you could comment on, you know, helping come up with new ideas. And really, that, that more creative side of things? Or do we still need the people asking the right questions and all of a sudden, though now you don’t need to go to a program or write a sequel or be an expert clinician in an area? But you can. You can kind of leverage this to help you execute an idea. How do you? How do you see those 2?
Divya Pathak: Yeah, I think that’s Ben, I think, Generator Vi plays a big role in both the content creation and the summarization. When it comes to at least health systems. Let’s talk about content creation, right? Specific to clinic clinical workflows. the next generation is an important administrative task that uses with generating clinical notes pre-authorization letters query, responding to patient queries, and also producing patient education material to support their care journey.
So I see that as an important use case when it comes to using generative AI, especially in its nascent stage. and that’s going to not just it’s going to improve the clinical improve efficiency when it comes to the clinical staff, but not necessarily remove the human. In the loop, there is still a requirement for the generator text to be reviewed by a healthcare professional before actually handing it out or signing off summarization on the other end. I think as Dr. Bala was mentioning around. Summarization of text involves condensing lengthy articles, and we talk about sometimes 300 plus pages of Pdf, that get actually faxed to a healthcare institution. how how we are able to synthesize and provide concise and easily understandable summaries.
I think generative AI has got another big potential and extracting key points, important findings, and relevant insights by reducing the amount of cognitive and clinical burden administrative burden and increasing patient experience, and also providing time for providing the support needed for the patients. So I see a big role of generative AI in both.
Ben Maisano: Yeah, though, that makes a lot of sense, just the efficiency that is possible you talk about. If the research side of anyone knows what physicians have to go through for kind of continuing medical credits just keeping up with the Times. There are these massive articles that the expectation is you keep up. And then in that day-to-day, understanding a full patient story, right? Could take you 20 clicks in an hour in the Emr verse. You know it. Can it be a lot more efficient? then that’s just the reading side to your point on all the writing and charting and notes that they do. But yeah, follow any thoughts on this.
Bala Hota: Yeah, I think the human in the loop feels to me also like a key component. because you know, in health care, it being as highly regulated as it is, and the stakes being so high, it adds that layer of accountability and safety, when you can almost frame these models as assistance to a clinical practitioner or someone else who’s doing the work and that, you know, they get the benefit of automation. but you also have the yard rails around safety. While I feel like, we learn more about the potential of how these can get used. And you know what the easy use cases are. so so yeah, these lend themselves really well to sort of being almost like a consultant or an in-assistant, you know, as you’re going through. So and that is, you know, very exciting.
Ben Maisano: Yeah. that that does seem like a real risk mitigator, as it’s still early. And we’re learning that this human is in the loop, our co-pilot you’ve seen. I know the programmers out there have been now using this co-pilot for all the coding assistance plugged into their ids. And you, if you’ve heard of the auto Gtp. Which is like you, can give it some goals. And it’s connected to the Internet and can go like on a schedule. 2 things for you. you’ve seen these versions out in the industry outside of healthcare co-piloting today. How it’ll be applied to clinicians or operators and healthcare is really interesting.
I think it gets to some of this need to mitigate that risk gets to explain ability in, in, in, in models. And so I was just playing around with the I always have like Chat Gtp and I have Bar and I have some others. But they all basically answered the same when, after I had a session with them. You could ask anything like what’s going on with the value is care in health care, and what’s the latest trend? It’ll spit out a whole beautiful article.
But then, you say, can you send me a source link for this? And they all basically reply, sorry, no, I’m not a search engine. And so that is where you really start to unveil some of the limitations of this of how they’re trained. It is a 270, you know gigabyte database of text a hundred and 1.7 5 billion parameters. I think I heard it. This huge synthesis of this is all the text of the Internet. And so you lose this barrier of some kind of source tracking, and they won’t provide you right now with links to answers or something like that. How? How have you dealt with explainability in the past, with maybe calming algorithms, whether it’s a simple logistic regression or other things that seemed explainable versus the neural net where it started to expose some of Hey, this is a black box, and that’s been a challenge in health care. What do you think about this now? Getting into generative AI regarding explainability and then tactics you’ve used in the past to deal with it
Divya Pathak: right? So I know that. you know. Explain. Ability is not a new problem with AI whether it’s machine learning or AI dealing with human reasoning. Black box models are strictly known when it comes to integrating them within clinical workflows. So the very first thing that we have established in the Center for digital help is actually to create deployment workflows with standardized development practices when it comes to building the metadata and provenance around each chip of the AI Development life cycle whether it comes to building metadata around the data that’s used. the motioning that’s related to required for the data the experiment itself being tracked across several data sets to actual models being cataloged and governed. there’s always that trust that needs to be established right from the development fees prior to the translation of those models into clinical workflows.
And the second thing we’ve done in the past and currently in practice is to establish a very standardized translation process which includes a multidisciplinary expert team review of these models with a certain level of accuracy that deems for performance to look at how they would actually be piloted in an in a clinical setting prior to actual use in production. So there’s a very, very big emphasis that’s put in place to support the clinical studies, the pilots, private studies that are required to test the actual use of models which is bridging the chasm between development and the real-world deployment and establishing a good level of documentation to show that it can be well used and at an acceptable user experience in the clinical workflows. So those are very important.
And besides that, Mlops is a very big function in understanding the shift when it comes to the performance of these models itself in production. being able to look at the drift in the data when it comes to any change in practices. If there is a data pattern that’s changed being able to monitor, being able to actually provide that level of proactive reporting of those details. So that we’re actually not. we are having better change management when it comes to retraining this model as being another practice. that’s been very established and standardized.
Ben Maisano: and that makes a lot of sense around kind of quality control, and the idea of metadata to kind of organize. At least you know how it was trained, or people have reference points to build trust in this that feels like it would help a lot.
Divya Pathak: We don’t want black box models with no reasoning behind it being actually even presented to the clinical staff. So payload to the existing outputs is another important thing that they got to be a payload that explains the features that actually were used in developing those models which can be dynamic in nature in terms of the weights and actual features themselves, but providing that level of details along with the model output as being one of the practices as well and well accepted. In fact, physicians are looking for the details of what are the clinical fields that have been utilized and coming up with those decision outputs.
Ben Maisano: Yeah, that makes a lot of sense. And it’s kind of like if you can even show the top-weighted thing in a given case. This is why we’re predicting the risk of sepsis in this patient. Here was the way to think. Yeah.
Bala Hota: it’s kind of interesting. To think there is no national model for how to deploy these kinds of you know, predictive modeling or or AI into clinical care. we tend to focus on use cases that are maybe more back office where automation is there. But there’s safety. You don’t have to worry about it. Patient facing from a safety perspective. I think the framework you’ve laid out is terrific, because, you know, if you think of a like a continuous Icu monitor, you know, like a telemetry monitor or or arterial blood, you know, blood pressure from an A-line. there’s
Bala Hota: a well-understood way that had been approved and how that got the clinical care and an expectation around it. Even clinical guidelines, I think positions have been trained to know how to look at the evidence like Cochran reviews and the level of evidence that this is a class one recommendation versus Class 3. And maybe this is a guideline. But this might be based on this one clinical trial. we’re not there with AI so that data literacy piece. Imagine if there was like your framework was a national framework right, and all positions as a part of their residency or Med school knew the 5 things to look for when they’re looking at an AI model, and in clinical care to even check. Is this an explainable model or not like starting with that? The hype is such that there are probably positions where we’ll just buy into the idea of AI working and not understanding the concept of failure, modes of these different things, and the level of vigilance. So so kudos, because, yeah, I think that’s how we get these to move beyond just back office to actual clinical facing algorithms that then actually, truly improve, you know, quality and outcomes of care in a safe way.
Divya Pathak: Got it. And to make this not just visible within Mayo Clinic but actually to establish some level of open standards we work in. We founding member of the Health of Coalition AI. This is an in it to the community of industry and academia which Mayo Clinic is a big part of in establishing those guard reels, and the metadata around clinical adoption of AI And in this realm our group is focused on establishing scientific eminence in this space. So we are actively publishing by collaborating with our other institutions to get some level of consensus around some of the metadata that’s gathered. And also Google has been a big partner we working with them to build those model cards that really explain the end-to-end development of a model just like how we would get it from the automotive industry when it comes to building a car. What does it take to build a model?
What is the outcomes? What are the inputs? What is the data distribution? What are the expected outputs being able to provide the business context, going back to the data literacy when it comes to. How is this model even used in a clinical workflow? What are the established metrics to determine the outcomes that are related to the model? So that’s something that we’re working on actively. So we established a scientific eminence that’s required for wider adoption of these standards.
Bala Hota: Yeah, you know, I, when you think about this, that’s excellent. Yeah, I think the automotive industry airlines is another one. Of course, in health care, we always look to like. If you think of the combination of the models plus the interface, how that is in these sorts of safety environments can have such a huge impact. you, you know, like, I think it’s the 7 37.7 37, Max. And just how it was that sort of connection between the algorithm plus the interface that led to their recalls. And they’re re-engineering. you know, we should be eyes open and health care, and how to do this the right way and put those frameworks in place.
Ben Maisano: Yeah, it’s great to kind of hear, like we have to raise the fluency in the room on being professional about these and knowing the limits.
Ben Maisano: you know, I think most people don’t know all the evolution of the Chat Gtp. Even like the first Gtp. One was like this open-source database, a very smaller model. Gpt. 2, was trained on Reddit articles that I had at least 3 likes. So that was the barrier of quality for them 3 likes on Reddit, and it’s it gets into the model. And now, obviously, it’s a much, much bigger set of all this Internet data. But it it’s still the Internet data. So it’s almost ironic what you’ve always heard in the past, hey? Don’t go to Dr. Google. You’re going to get the wrong information. You’re going to worry. And now everyone in health care is looking at. How do we use this thing, which is large, even train of basically the Internet where you said told people don’t go. So I think it kind of leads to the standards and understanding you guys are talking about.
Ben Maisano: how do you view getting to the next level of, do we actually need healthcare, trained Lms, and when you, as you look at some ideas and we’ll talk about ideas in a second that you’re thinking in this area. What’s the framework for those ideas? But can you? Can you go to just prompt engineering, and seems like, maybe you can do that and kind of stand on the shoulders of these giants? Or do you need to actually develop our own healthcare? Lms, how do you? How do you see that playing out?
Ben Maisano: and any ideas like how you the levels of needing to do this yourself from scratch in a more controlled way for health care or building on top?
Divya Pathak: Yeah. I’ll take a stab at it. Then. we’ll talk about these models, at least the Gp, T, 3, and 4.
There’s a lot of information from the Internet that’s got into it. So it’s got a lot of depth and personality when it comes to its responses, but necessarily doesn’t fit the complexity of health care when it comes to domain-specific nuances
So we have to train it on clinical data and Taylor to specific healthcare settings so that we can start to solve some of the challenges. That’s only domain-specific. for example, I think Dr. Bala was talking about ontologies and terminologies, healthcare involved so much complex terminologies and unique conceptual understanding that’s needed. So training and large language models.
That’s all healthcare, specific knowledge and medical information can generate more accurate insights compared to these generic models, and also addressing biases and ethics ethical concerns in healthcare which is a huge focus when it comes to the adoption of AI health care data is inherently biased due to the humans involved, and the system challenges so ethical considerations and biases need to be dealt with differently when it comes to health care compared to other domain-independent or domain-specific industries and being able to identify those biases meeting anything.
Those biases are specific to healthcare. A ha can only be done when it’s a model that’s trained on health care data the last one is the improved performance of healthcare tasks as much as we can have fine-tuned models by employ by adopting prompt engineering on top of existing models. medical diagnosis when it comes to diagnosis and treatments, being able to be accurate because there’s no room for error in this kind of AI algorithm and results. supporting clinical decision-making requires a higher level of accuracy than what exists.
What exists today? So I would say that even though there is a good level of advantages when it comes to prompt engineering on building on top of these existing large language models. in order to really see the promise of these models in healthcare. We’ve got to really start looking at building that data, sharing, and collaborations to provide that level of volume when it comes to training his healthcare-specific models.
Ben Maisano: Yeah, no, that’s a good point is, people don’t. I don’t think, appreciate how large of a day they need for this thing to get fluent and seem like it knows any topic. And is, you can ask it anything. that’s a hard data set to compose even with history. yeah, that makes sense. it’s in a ball. I know we’ve looked at internally all the tools we have at our disposal. You’ve seen. Aws come out with bedrock to kind of build stuff. They plug stuff into the sage maker, certainly Azure with their open AI studio. I think Google’s the only one I saw that had their mid palm to is actually health care, I guess. The specific model that came out. How do you all? yeah. But any other tools you want to just share, or the things we’re thinking about, how to how to kind of execute on some ideas. Yeah, I mean. And we’ve been thinking about this from a product development perspective. for sure, I think
Bala Hota: globally, for when you’re talking about areas where Phi is not a concern, or where you get there some of the regulatory aspects where it’s it’s easier to handle. this whole co-pilot assistant sort of interface really feels like the right way to go particularly around automation, or maybe on the safety dimension. You with less concern. The one thing is, and you kind of mentioned this. When you look, there’s a lot of practice variation in health care. When you start looking at the clinical side, it can be a provider-specific specialty, a specific region, specific hospital specific.
And we’ve got this kind of interesting opportunity right now, how much do we want to standardize nationally in our products and say, this is the one pathway versus how much do we want to allow individual variation that is acceptable, and allow retraining almost, or configuration at a single provider or group level. And that tension is a is one. You know. We’ve been thinking about this a bit because you can imagine you roll out a nationwide documentation improvement initiative that is AI-based and individual hospitals will have their own kind of implementation, their own policies, and procedures. You want to really be able to account for that and not impose so that it is from an Llm perspective. That’s more evidence around. you know, focused, retraining or transfer learning, or having your own sort of individual small data set that you can refine the larger model and interfaces that can account for that. So so I think you know, as we think about products.
For now, with what’s out there, we’re very much thinking about this assistant model like I need an expert assistant. I can quickly get it. Look up these guidelines, these pathways, this an expert advice. And then it provides me with that information.
And I think, some of the natural languages interfaces to data so interrogating your data almost like it’s a person, you know, and you can ask the questions around. Tell me about this data set, and what are the trends? That’s that starts to really democratize the insights in a way that You know, I know most of my career I’ve been looking for so that could be really exciting. So I think those are the 2 big areas that I know we’re getting really excited about as we think about the product.
Yeah, that it’s it’s definitely interesting to think about the breakdown and not needing those rigid dashboards and the hundreds of reports every health system has. And then you need to tweak it. And you need to tweak too. And it’s this ticketing and changing, and I know by you servicing that world for years. and that’s every health system I’ve ever seen. They have tons of these reports that they constantly need. Just first, I think what you’re describing is a world where you can just talk to an interface, and it’ll on the fly come up with the exact report you want. That is a really enticing kind of area. If you have any exciting areas you guys are looking at for this. And how are you thinking about kind of organizing ideas, and which ones feel tangible and close to home? For now versus kind of a bit off
Divya Pathak: can support complex decision-making at a broader scale. But it will likely take years before all the technical, ethical, and legal challenges and considerations are in place to make that vision a reality. while that work is in progress. And it’s an active area and research. we do think there is plenty of productivity related to use cases which is more in the nonclinical areas to tackle the back office as well as promising opportunities to plan to streamline the clinical process. That leads to better efficiency when it comes to the overall workflows. So really, law, nonclinical back-off is used. Cases are the ones that we’re looking at. And one thing that is actually, we have much closer is the clinical documentation burden when it comes to providers. I read in one of the articles where it was.
It was mentioned that physicians are the most expensive data entry professionals in health care. when it comes to reducing talk, that event clinical burden, so that we improve the patient experience. That’s been a very important topic, especially when it comes to digital health initiatives. and we are working very closely with Microsoft on their nuance BA, which is recently integrated. The Chat GPS opens AI services initially, the da. It’s been introduced, I believe, in 2020 and used to have a human review in the process. it’s an ambient listening solution that listens to the patient and provides a conversations and is able to generate the text and the clinical notes that then goes for a human review to sign off before it’s actually added to the EHR. So there’s so much good work that’s done in my by nuance, and actually making that process even much more seamless.
There’s very little information that needs to be reviewed by humans, which is actually going to improve even further when it comes to reducing the documentation burden by declination. So that’s something that we are looking at already. And just as Dr. Bala was mentioning a conversational AI, that’s another piece we’re looking at. How do we build virtual assistance to support patient engagement and patient education hen it comes to digital health platforms? being one of the key focus areas. I’ll be able to build these conversational chatbots that mimic what Gpt does today but are very specific to the patient engagement use cases. which really doesn’t get into the legal and the ethical challenges as much as when you’re dealing with Chat Gpt to your generative AI, that’s helping with diagnosis and treatment. So that’s really clinical on the bit side. So we’re trying to really shy away from that till there is a little more maturity when it comes to dealing with all the interpretability and trust issues. we generated. AI,
Ben Maisano: yeah, that makes a lot of sense. I do think it’s gonna find these use cases that are safe to experiment with and learn. And yeah, I heard someone recently say to when there’s a lot of challenges against the and the lack of trust. He’s like, what did you train your humans on? And I think there is some kind of you know. understanding and realistic that we have to be like humans and make mistakes. Stay, there are inefficiencies, I must say so. How much better does the A have to be than the average human before you train? And there is that high standard for something new like this but it’s not ever going to be perfection. We don’t have it today, and I don’t think we’ll have it with any tool. but it’s navigating those waters to kind of figure that out.
And a lot of cases we’ve we’ve heard is are you keep that sanity check in the middle where it is like this advanced spell, check, and generator. Someone’s looking at it before the output gets committed. Whether it’s back to the patient, to the Admir. And I know today it’s like how accurate is spell check, or how accurate are these little suggestions. If I start typing in my Gmail and it puts it out there a lot of times. It’s right, but I’ll tweak it, and it doesn’t need to be perfect because it’s understood the interface completely de-risk it right because I’m still in control. It’s just made me a lot more efficient.
And in a similar way. it sounds like, that’s a lot of this kind of efficiency plays with patients. and it’s also not like these. Things don’t exist today. There’s a lot of rollout of bad chatbots out there already. Some virtual triage, and as soon as there’s any ambiguity still kind of steps up to a human queue somewhere, right that that parsing and de-risking has already been out there. So you talk about the Schmidt Thompson protocols that’s gonna ask you like these 40 to 60 rigid questions to try to triage you, or a nurse is going to do that live. And then you still kind of just get to the next level of care. this feels like the potential to at least be a level up from those to start with.
Well, thanks, guys, for kind of going over this whole industry. And what’s going on? If you have any closing thoughts on when we do have trust what? What was it really exciting thing, or what? Your biggest kind of hairy question out there, or even want to share how you’ve played around with these just to learn about them for fun. any closing thoughts?
Divya Pathak: Yeah. Then, I think when talking about generative AI. I was very excited to really look at it as the next Spotify when it comes to health care honestly. just the way the retail businesses have actually excelled in the way they market personalized and provide that seamless customer. Experience. besides, generative AI supporting the providers. I see a big leap when it comes to supporting the patient experience. whether it’s by adopting those data-centric approaches, streamlining appointments being their co-pilot when it comes to their care, journey at a particular health system, providing tailored treatment plans and recommendations, and enhancing that communication between the health system and the patient itself. I I see a big promise when it comes to generative AI. So my way of actually playing with you. The Chat Gpt is asking some questions that often get asked when it comes to clinical decision-making.
And it really a lot of questioning around the medical literature to see if I’m able to get the answers that I would get from a physician. So it’s a human clinician response versus chat Gpd’s response. And that’s been the way I’m actually playing with it.
Nice. That’s great. Yeah, Bala. any?
Bala Hota: Yeah, I mean, I’m of 2 minds. I think, from a clinical perspective. It’s not even a technology. It’s the. It’s the regulation. It’s the liability. It’s the accountability we haven’t solved. For there, there was that interesting article that recently came out that said that you know, a chat Gp, I think, was more empathic in its responses than positions, right? And it makes you for a minute think, wow! That’s incredible. Imagine, if you have this sort of agent that’s automated that can provide that empathy, empathy, and communication. That’s better than a physician because physicians are human beings or press for time. There’s, you know, all these other constraints. Can we say that a machine is, it has empathy?
And so at some point does it. Does a patient actually receive empathy from a machine And so there’s that whole piece of it which I just think I can’t. I don’t know. Somehow I keep dwelling on that idea of what is empathy. And then the other thing I’ve been thinking about a lot is just the regulatory framework, you know. If a mistake is made. if the Tesla autopilot makes a mistake. you know. it’s always the human driver right now. We haven’t figured out how to have that liability conversation without the human in the loop. And so I think that’s going to take a while, I think in terms of So I’m just. I’m following the space closely in that regard. I I I think in terms of how on, you know, email search is always a big challenge for me.
Bala Hota: So I keep every email. So the thing I’ve been playing around with is that can I talk to my email and a chatbot, for, you know, sitting over my email to find things. So that’s you know, playing around at home. That’s the hobby. So you know, it’s exciting when you start thinking about it that way, like as that assistant who can make things easier.
Ben Maisano: Yeah, no, that’s really great. And that that article you reference was very interesting where you put side by side right. The Chat Gp. Response to physicians, and were all more concise, and chat types were kind of more formal In reality, that’s the physician’s job is, to be efficient bouncing from room to room but getting to the point. Reduce the noise where Chatgpt can be very formal, and sometimes a little vanilla. It’s is it lacking personality? So I found people use it as what an answer should look like almost like a reference point to make sure you’re not missing anything. But it’s not the actual answer, right? It’s like this generic. very thorough reference to guide seems to be a lot of around. No, that’s good.
Yeah. Our favorite kind of game to play once a week. Now, around the dinner table is, pick a topic that our kids are in like a sport, and have it generate a little wrap. And then, you know, the nice thing is like, sometimes it’s kind of soup, too, Corny, and you could just say, Hey, make this edgier, and it’ll refine it. And then the kids read it at the table. And it just it’s just so impressive in that way of how you can manipulate a language and come up with things on the fly. So certainly very promising. But thank you both very much for the time.
I’m thinking about how to apply to technology, to health care and some insight, and how you think about it. to appreciate that and maybe we’ll do a follow-up session in another 6 months that the pace of the industry moving is so rapid that I feel like, if this even holds a test of time for that long, it’ll be good, absolute pleasure to be here and thank you for inviting me, and nice to meet you, Dr. Paul.
Bala Hota: Nice to meet you, too.
Ben Maisano: Okay, take care, guys.
Divya Pathak: bye, bye.