Understanding Data Observability: How it Enables Organizations to Deliver Value Faster Than Ever
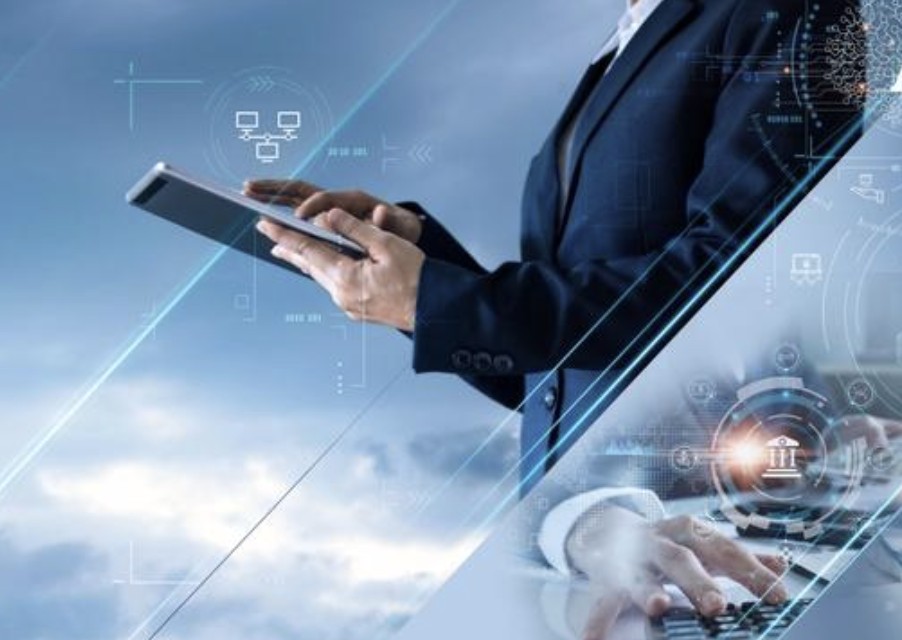
By John Scutz, Adeptia Inc.
Data drives today’s digital transformation era. Expert estimates predict that 2.5 quintillion exabytes of data are generated daily. Now, that information normally resides in diverse systems, including cloud-based, on-premise systems, and hybrid ecosystems.
The information includes streaming data from IoT sensors, smart devices, mobile trace data, and more. Being so diverse in nature, the chances for the presence of errors or discrepancies in this data are also high. As per Harvard Business Review, nearly half of newly created data encompass an error. As a consequence, 84 percent of CEOs doubt the integrity of the data on which their decisions are based.
Further, because systems and data sources are more connected than ever, the interdependency between systems leads to newer problems. These systems get more complex and if any change happens in one place, the ramifications can be devastating. For instance, a data pipeline that’s broken a data pipeline might bring all the systems to a halt, or it could trigger dashboards to fail, reporting incorrect KPIs to the top management.
These challenges can be resolved using a foundational element of data operations (DataOps) called data observability. Data observability is a process with the help of which organizations can monitor and attend to the quality as well as the availability of an enterprise as it is moving. Data observability enables organizations to extract deeper insights into what is happening with data and why. Data observability uses real-time machine learning intelligence and helps users detect outliers and anomalies in the data as it traverses throughout the enterprise.
This blog post explores data observability, its significance on the health of a business, and how it enables organizations to deliver value.
Understanding Data Observability
Data observability enables users to identify, troubleshoot, and resolve data issues in real-time. These platforms automate data monitoring, tracking, root cause analysis, triage alerting, and service level agreement (SLA) – including data reliability. Organizations rely on data observability platforms to extract actionable insights, develop machine learning systems, and facilitate innovation. Using these solutions ensures that all the tasks related to data management are standardized and centralized across systems for an uninterrupted view of issues and impacts across the organization.
Data observability includes the following steps:
- Perform discovery, monitoring, and profiling: Users collect information about where data resides. This information entails, what it contains, and who uses it, and then monitors that information in a proactive and continuous manner.
- Analyze information: Processes information with the help of enterprise data, assesses historical trends, and detects outliers – all with the help of AI/ML for automated intelligent analysis.
- Execute Visualization and Alerting: Provides primary users with the help of dashboards to visualize real-time tasks and provide alerts that alarm users of outliers and help them make decisions.
Importance of Data Observability
Organizations need to trust in their data. They use analytics to recognize strategic opportunities, support line-of-business users in helping organizations make tactical decisions and feed AI/ML models that automate day-to-day tasks.
Data observability plays an important role in ensuring that data are trustworthy as well as reliable, enabling organizations to deliver value by:
- Ensuring trustworthy data for accurate reporting and analytics. By recognizing anomalies and automatically alerting the appropriate users to potential problems, the data observability platform empowers organizations to be proactive instead of reactive, addressing data problems that have the potential to disrupt business operations and create expensive problems.
- Reducing costs and time to resolution for operational problems. Data observability provides information that helps users determine the root cause of an issue. That means solving problems before there could be bigger problems.
3. Eliminating risk and facilitating successful transformation initiatives. Digital transformation is a top priority for a variety of businesses, but it inevitably involves more data and more rapid change than ever before. Data observability helps data analysts, data engineers, and other users with a useful understanding of what’s happening to your data.
4. Enhancing the Quality of Data: Data observability platforms help organizations detect and address data quality proactively. These solutions can detect problems, inconsistencies, anomalies, and errors in the data lifecycle, preventing potential downstream impacts and ensuring that data remains reliable and trustworthy.
5. Improving Data Reliability: As organizations get comprehensive visibility into data pipelines, they can ensure that data is available, reliable, and consistent. This reliability is important for different applications that rely on real-time data, including supply management tools, financial trading systems, and customer personalization engines.
Conclusion
In the current digital transformation era, where data is complex and diverse, leveraging data observability platforms has become a must. Not only do these advanced AI-powered solutions enable organizations to manage data properly but also deliver the value promised to their customers.