DeepSeek: A Milestone In AI Efficiency, But Not The Agi Breakthrough We’re Waiting For
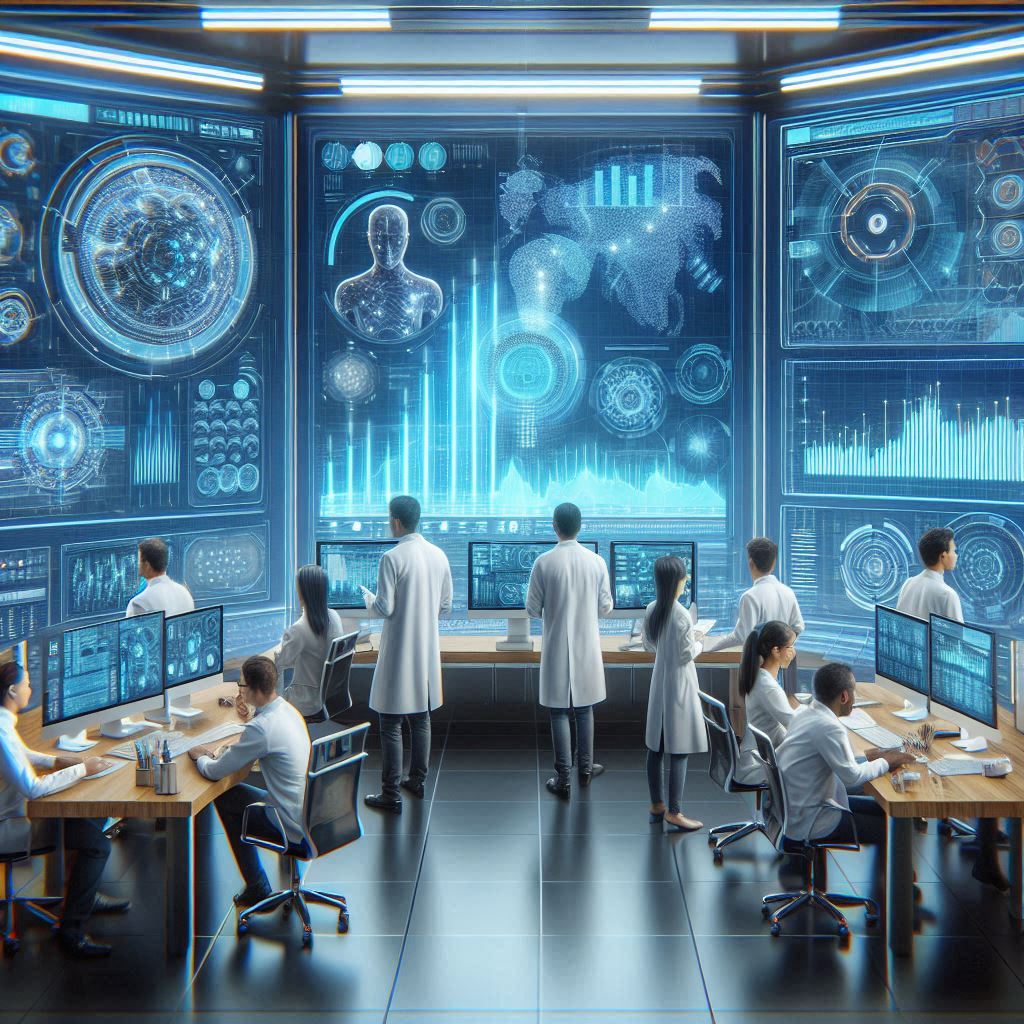
By Matthew Ikle, Chief Science Officer of SingularityNET, the world’s first decentralized AI platform.
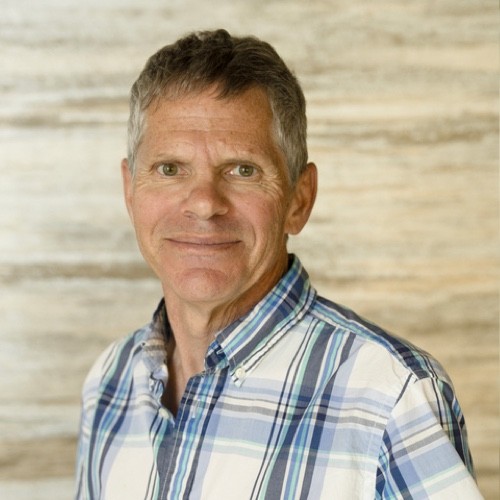
DeepSeek has generated considerable excitement, but much of the reaction seems to misinterpret its true significance. While it represents an impressive leap in efficiency for large language models (LLMs), it doesn’t mark a breakthrough towards artificial general intelligence (AGI) or a fundamental shift in the landscape of AI innovation. Instead, it represents a rapid acceleration along an expected trajectory – a notable engineering achievement, but not a paradigm-shifting event.
Technological progress often follows a predictable pattern. In the 1990s, high-end graphics rendering required supercomputers. Now, smartphones can handle the same task. Similarly, facial recognition, once an expensive niche, is now a standard feature. DeepSeek fits into this broader trend; the breakthrough isn’t surprising in its nature, but rather in its speed. Those familiar with exponential technological growth will recognise that, as we approach the technological singularity – a theoretical future event at which computer intelligence surpasses that of humans – the pace of innovation will only increase. DeepSeek is one of many examples of this unfolding trend, reflecting the accelerating growth of AI technologies.
At the heart of DeepSeek’s innovation is efficiency, not a radical new approach to AI architecture. Its use of the Mixture of Experts (MoE) model is a refinement of a technique that has been around for years. The key difference is that DeepSeek activates only 37 billion of its 671 billion parameters at a time, which reduces computational costs by 1/18th compared to traditional LLMs. Other optimisations, such as reinforcement learning to enhance reasoning and multi-token training to improve efficiency, allow DeepSeek to significantly outperform competitors like OpenAI and Anthropic in terms of cost. This is a major achievement in engineering, but it does not represent a breakthrough in AGI development.
A particularly significant aspect of DeepSeek’s strategy is its decision to release its model as open-source. This move contrasts sharply with the proprietary approaches of companies like OpenAI, Anthropic, and Google. Open-source AI promotes faster innovation, broader adoption, and collaborative improvement. Beyond its philosophical stance, DeepSeek’s decision is also a strategic business move. It signals confidence in a model based on services, enterprise integration, and scalable hosting. This open approach also provides the global AI community with a powerful toolset, helping to reduce the dominance of American tech giants and fostering a more decentralised AI landscape that aligns with the open-source AI movement.
DeepSeek’s breakthrough coming from China may surprise some, but it shouldn’t. China has been investing heavily in AI research for years, and this isn’t the first time the country has taken a Western innovation and rapidly optimised it for efficiency and scale. Rather than seeing this as part of a geopolitical contest, it’s more constructive to view DeepSeek as a step towards a more globally integrated AI ecosystem. The future of AGI is more likely to emerge from open, collaborative efforts than from nationalistic silos. A decentralised, global approach to AGI development would ensure that AI benefits humanity as a whole, regardless of national borders.
While much of the attention around DeepSeek focuses on its impact on LLMs, it’s important to think beyond that. LLMs, as powerful as they are, are not the path to AGI. They lack key AGI characteristics, like grounded abstraction and self-directed reasoning. If AGI does emerge in the coming decade, it’s unlikely to be based purely on transformer models. Alternatives, such as neuro-symbolic AI, could play a more essential role in achieving true general intelligence, offering new avenues for innovation.
As DeepSeek’s efficiency gains accelerate the trend of LLMs becoming a commodity, investors will likely shift their focus to the next frontier of AI. This could mean more investment in alternative AI architectures, decentralised networks, or new hardware solutions like neuromorphic chips. The commoditisation of LLMs could signal a broader shift away from traditional models and towards more diverse, innovative approaches to AI. This opens the door to exploring non-traditional AI solutions that could be more aligned with the development of AGI.
Decentralization will be a crucial factor in the future of AI. DeepSeek’s innovations make it easier to deploy AI models in decentralized networks, reducing reliance on centralized tech giants. This shift could facilitate the rise of AI ecosystems that prioritize privacy, user control, and interoperability, like those being developed by organizations such as SingularityNET and the ASI Alliance. A decentralized AI future would foster a more democratic distribution of power, challenging the hegemony of tech giants.
While DeepSeek represents a major milestone in AI efficiency, it is not a step towards AGI. It’s a significant acceleration along a known path, one that pressures established players to reconsider their business models, makes high-quality AI more affordable, and highlights China’s growing role in AI development. Ultimately, if we want to achieve AGI, we must look beyond optimizing today’s models. We need to explore new architectures, foster open collaboration, and ensure that AI development remains decentralized, global, and accessible to all.