The Pathway to Accelerating AI Adoption – Empowering the People
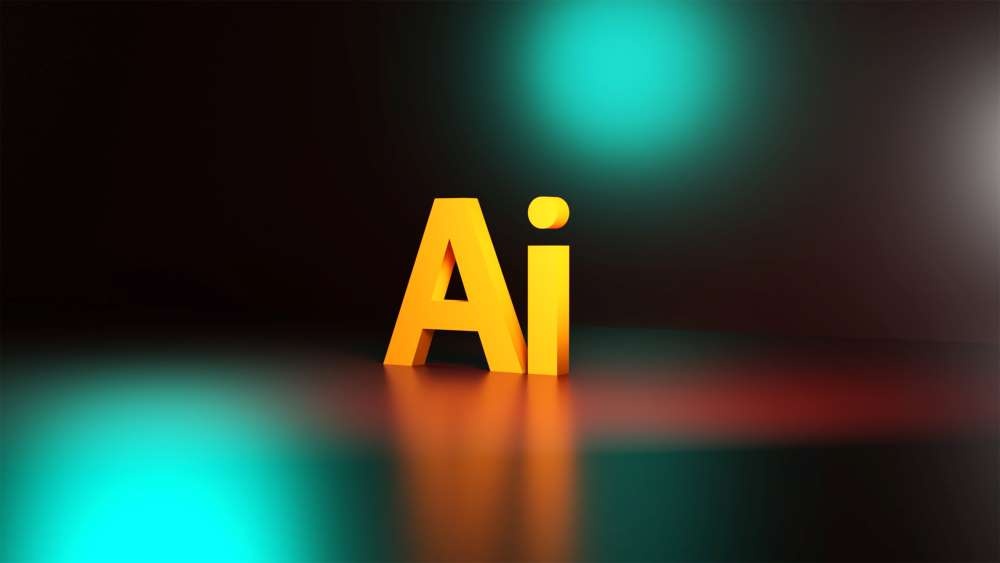
By Brian Backer, MBA, Certified Analytics Professional
What can a software application that came out in 1985 teach us about the path to accelerating AI adoption in 2022 and beyond? The future of AI is a hot topic — many hail it as the single greatest cheat code for modern life, while others express concern for its potential negative impact on workforces and economies. But what if it’s more practical than that? What if AI has the power to enhance human output rather than replace it entirely? And if that’s the case, then how and when do we get there?
Last month, I wrote in The Pathway to Accelerating AI Adoption that the number of scarce resources necessary to apply AI to a single task was the biggest inhibitor to AI becoming more ubiquitous in a practical business sense. I shared how Google Research has introduced a high-level architecture for tackling some of the major limitations constraining broader AI applicability, and this line of research by Google and others is going to be critical for many companies to begin meaningfully adopting AI and machine learning at scale.
But even with meaningful progress in AI applicability and being able to apply an AI model to multiple tasks, there are still a couple of other, perhaps more fundamental constraints, to exponential growth in AI adoption.
One which I touched on in the last piece is the scarcity of resources to aid in data preparation, cleansing, and integration. There are a number of buzzwords out in the marketplace including Data Federation, Data Meshes, Data Fabrics, and a plethora of content around each, but central to each of these is the goal of creating a centralized, integrated, governed, readily-consumable set of data assets in support of the business. In a sense, they all aim to productize data as a core building block for analytical and other use cases.
Perhaps in a future post, I’ll catalog some of the more practical, valuable content in this area, but in this piece, I want to focus on what I believe to be the more challenging constraint to broader AI applicability — the difficulty in deploying AI and ML into business workflows.
Adaptable models will attract developers to AI/ML use cases
It seems these days that every software developer is looking for ways to embed AI applications within their user experience to enhance user productivity, task efficiency, etc. Enabling this is some fantastic AI and ML as a service capability from the big public clouds including Microsoft Azure, Amazon Web Services, and Google Cloud Platform.
For teams fortunate enough to have skilled data scientists and machine learning engineers, public clouds offer services such as AWS Sagemaker and Google Vertex AI to train and deploy models natively in the cloud. In many cases, developers of software involving computer vision and speech can leverage services such as Azure Cognitive Services, AWS Rekognition, and Lex, and many more simply as APIs without requiring a machine learning expert or deep knowledge of how those services work. For smaller companies, this is game-changing, as they now have the ability to leverage models developed by some of the best researchers on the planet via a simple API call.
As AI architecture evolves to enable models to support multiple tasks, the applicability of such AI as a Service solutions will expand and even further empower developers without deep knowledge of AI or ML to leverage their power within new applications and apply them to new use cases.
But this is only an incremental step in the ubiquity of AI adoption…
The scarcity of software developers is the next major constraint
To really put AI adoption into overdrive, these types of AI services need to be more accessible to business users. Similar to the scarcity of data science and machine learning talent we discussed in the previous article, there are similar challenges in finding developers to bring those AI solutions to production.
Digital transformation is driving unprecedented demand for developers. Microsoft expects 500 million new apps to be built in 5 years, more than the number that’s been developed over the last 40 years. According to Charles Lamanna, corporate vice president of the citizen applications platform at Microsoft, “If that’s true, 450 million have to be built with a low-code tool.”
“There is a 1 million developer shortfall in the U.S. alone, and all these companies are struggling to create content and applications to go truly digitally native.” — Charles Lamanna, Microsoft
Cognizant Technology Solutions CEO Brian Humphries has also publicly recognized the impact of this gap between growing, pandemic-fueled demand for digital services and the shortage of developer resources to deliver them. He recently told the Associated Press “there is a demand level, unlike anything we have ever seen in many, many years. And that demand increase is ultimately fueled by digital acceleration. And every company in the world, regardless of the industry, is pushing toward digital business models. So that is ultimately leading to this situation that is faced today.”
So how do we solve this?
What citizen data science tools are aiming to do for ML development, low code/no code seeks to do for application and automated workflow development. Low code/no-code tools enable business users to build an application, using largely drag-and-drop tools and simple-decision making logic without the need for expensive developer resources. For the purposes of automating many business workflows, these tools can meet or closely fit business requirements in days rather than months and at a significantly lower cost.
There’s definitely still a distinction between low-code and no-code. Low-code tools still typically require some technical expertise to build an application but can be incredibly valuable simply by making developers and other technical resources more efficient at automating a workflow or developing a proof-of-concept application. No-code tools use primarily visual interfaces and typically only require a few days of training for non-technical workers to get trained on in order to be productive at building applications. The latter is the goal many technology providers are striving for and starting to see early success with.
Most often, the types of tasks that low-code/no-code is traditionally best fit for are not massive-scale enterprise systems but more specific to certain functions or even individual professionals’ workflows. With the significant amount of time and investment required to build an AI/ML model, these tasks don’t warrant the scarce time of a data scientist or machine learning engineer. But broadly applicable, multi-task models that can easily be integrated into a drag-and-drop workflow lower this barrier significantly and put AI into the hands of the business user.
This might seem far-fetched, but consider the introduction of Microsoft Excel, which puts the power of complex computations formerly done by custom code on mainframe computers into the hands of business users. How ubiquitous have Excel spreadsheets become, often using custom formulas in order to perform specific tasks or workflows? In many ways, Excel was the original “low code” software that replaced decades of custom-built number-crunching applications. In this light, you can almost think of low-code/no-code tools and AI as a Service as giving the same self-serve analytical capability that Excel gave to number crunching and intelligent workflow automation.
We are only beginning to scratch the surface of AI adoption
Hopefully, frameworks and tools such as Google’s Pathways deliver on the promise of helping AI models generalize beyond a single task, which will lead to dramatic increases in model applicability to new tasks.
Combining this with the adoption of low-code/no-code tooling that will put these more capable models into the hands of more business professionals could unshackle AI from the domain of a few to a capability builder for all business users.
And when this happens, we’ll see exponential growth in AI adoption, and AI will become a universal, nearly essential, tool in business decision making and workflow automation.
About Brian Backer:
Brian Backer is currently Senior Director of Analytics at LiveVox. He is a Certified Analytics Professional and holds an MBA from Georgia State University. Prior to LiveVox, he led customer analytics for UPS and has consulted several Fortune 500 organizations on insight-driven customer experience transformation while with the CFI Group.