The AI Discrimination Conundrum: What Life Insurers Need to Know
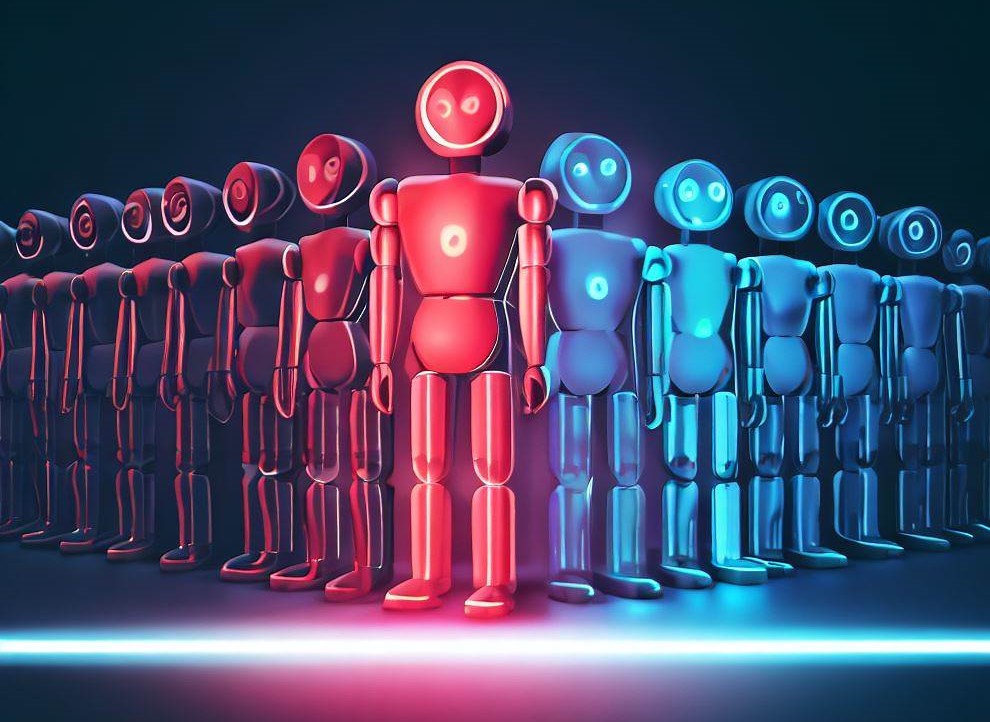
As artificial intelligence (AI) continues to permeate our lives, one thing that we’ve learned is that, because AI typically learns from human-created content and information, AI can unfortunately reflect the attitudes and biases of the society that gave rise to that information.
Efforts are already afoot to help address the potential discrimination that could arise from AI systems trained on the contributions of imperfect humans. Recently, Colorado officials proposed new regulations that aim to tackle the potential biases that can emerge from the use of AI in the insurance sector. This marks the first time a state government has attempted to provide specific guidance on the evolving practice of AI-driven underwriting. If passed, these regulations will require more in-depth risk assessments and compliance from insurers and could serve as a framework for future nationwide policing of discrimination in insurance.
However, for life insurers, the issue of AI discrimination is not as clear-cut as it might be for, say, property, or auto insurance. This is because life insurance underwriting is, almost by definition, a discriminatory practice, at least in the larger sense of the word. Insurance applications from lower-risk individuals are typically favored over those of higher-risk individuals, and healthier individuals are preferred over unhealthy ones. The prevailing view within the industry is that this form of discrimination is acceptable and necessary.
Yet in today’s world, the definition of acceptable discrimination is a constantly moving target. Unfortunately, risk factors in insurance can correlate with things like race and socio-economic status. And while it might make sense that an insurance company would take health considerations into account, the results can often mean an unintentional bias against certain racial and economic groups. And therein lies the problem.
For life insurers and their tech teams, tackling the AI discrimination issue must become a top priority, especially as more AI tools become integrated into the underwriting process.
Getting ahead of change
There’s a Peter Drucker quote that I’ve always liked: “You cannot manage change, you can only get in front of it.” In other words, if life insurers wish to prepare for nationwide regulations on AI-driven underwriting, then they need to get ahead of the politicians and adapt now. Because, make no mistake, the Colorado regulations are just the beginning. Other states are bound to start recognizing the issues with AI discrimination in underwriting and start to take action.
Fortunately, as I learned from when I was in politics back in the ‘90s and 2000s, businesses can always move faster than bureaucrats. So, take advantage of that by getting under the hood now before the politicians can. Begin by conducting a comprehensive assessment of your current underwriting practices. Odds are, you’ll be confronted with the main issue that lies at the heart of insurance: how do you spread the cost of risk?
In theory, you could follow the ACA health insurance route and spread the risk per person regardless of personal risk factors. However, that’s unlikely to please the clientele of a private life insurer, as it means higher premiums for everyone. That leaves you with the only option of adjusting prices based on personal risk factors. In other words, you must discriminate. But based on what factors?
“Discrimination” versus discrimination
Of course, on a certain level, we’re dealing with a semantic issue here. “Discrimination” can refer to the act of differentiating one item qualitatively from another. You know, this flat-screen TV is better than that one. But since the Civil Rights movement, the word has also taken on a negative connotation, referring to the act of segregating racial populations and treating them differently.
And people can get really touchy when it comes to health and insurance. I could put several random people in a room right now and start an argument over the following question: Should a person’s health status influence their life insurance rate? Some will say, “No, we shouldn’t discriminate based on health,” while others will counter with, “Yeah, but the guy who’s not smoking or drinking shouldn’t have to pay for the guy who is.”
This is what I’m getting at when I say that discrimination is not in itself a bad thing. The problem is that in the current political and cultural climate, discrimination has become a rather dangerous word. So my advice to any insurer is that before committing to rooting out any unfair discrimination in your underwriting, first try to define what you can discriminate against.
Poor health and lifestyle choices are clear examples of things that life insurance should discriminate against. It’s in the very nature of life insurance that better rates should go to people with better health outcomes and those who take better care of themselves. The challenge lies in making sure that intractable social issues and biases don’t inadvertently become confounding variables.
More data, not less
Obviously, any discrimination based on race, gender, creed, or sexuality is unacceptable. But here’s the thing. An AI algorithm will base its decisions on the data that’s been provided to it and, again, that data comes from humans who have both conscious and unconscious biases. This is the AI discrimination conundrum in a nutshell: AI learns from humans and humans are flawed. And it’s that challenge that AI developers are still trying to solve.
Out of the many proposed solutions for AI discrimination, one that has gained some traction is removing group data indicators such as race, gender, and sexuality from the training data. The idea is that if the algorithm can’t “see” these factors then the result will not be discriminatory. However, this still runs into the problem that if the training data is one-sided to begin with (e.g., more men than women apply for life insurance), then the algorithm will still find other factors that can induce a bias. And the fact that certain population groups having poorer health outcomes is endemic to a discriminatory society will continue to affect the underwriting results, even with those group indicators removed.
Instead, what’s needed is more data, not less. Think of it this way, a human underwriter will know that factors such as race or gender don’t matter when determining an applicant’s risk level. However, because an AI algorithm lacks the cumulative experience of a human, it can easily double down and accidentally discriminate when it doesn’t have enough data. The solution then is to provide your AI algorithm with all the data you have on an applicant, including race and gender. Why? Because the algorithm will then be able to learn that these factors are irrelevant.
This was highlighted in a recent study that considered a fintech lender that uses an AI algorithm to decide whom to grant a loan to. The researchers found that including gender data can significantly reduce discrimination by a factor of 2.8. This is because, without access to the gender data, the algorithm would overpredict female applicants to default via proxy variables such as profession or work experience, and these proxy variables would historically favor men. But by including gender data, the algorithm could correct its predictions.
Final thoughts
In summary, I don’t expect the AI discrimination issue to go away easily. AI, for all its much-vaunted power, is still a very new technology and it remains to be seen whether it will live up to all of its promises.
With that in mind, life insurers and their tech teams will need to continually test their algorithms to ensure there’s no unfair discrimination. That’s something that will become even more important as state governments continue to roll out regulations on AI use in underwriting.

Bob Gaydos is the Founder and CEO of Pendella where he leads a team of innovators in the insurance industry, automating the underwriting process through AI and big data. Over the last 10 years, Bob has founded, invested, advised, and operated innovative companies in the benefit & insurance industry, such as Maxwell Health: an online benefits administration platform acquired by Sun Life in 2018. Connected Benefits: an online insurance agency acquired by GoHealth in 2016. Limelight Health: a group underwriting platform acquired by Fineos in 2020. GoCo: an online platform for HR, benefits, and payroll. Ideon (formerly Vericred): an innovative data services platform powering digital quote-to-card experiences in health insurance and benefits.